Ensemble methods combine multiple models for better performance from "summary" of Machine Learning by Ethem Alpaydin
Ensemble methods are a powerful approach in machine learning where multiple models are combined to achieve better performance than any individual model. The idea behind ensemble methods is that by combining the predictions of multiple models, we can reduce the variance and bias of the overall model, leading to more accurate and robust predictions. There are several different ways to combine models in ensemble methods. One common approach is to train multiple models on different subsets of the data, such as using different features or different samples of the training data. These models are then combined to make predictions, often by taking a weighted average of their individual predictions. Another approach to ensemble methods is to train multiple models using the same data but with different algorithms or hyperparameters. By combining models that are trained using different approaches, we can capture a wider range of patterns in the data and improve the overall performance of the ensemble. Ensemble methods can be used with a variety of machine learning algorithms, including decision trees, neural networks, and support vector machines. One popular ensemble method is the random forest, which combines multiple decision trees to create a more robust and accurate model. Ensemble methods are particularly useful when dealing with complex datasets or noisy data, as they can help to smooth out the noise and capture the underlying patterns in the data more effectively. By combining multiple models, ensemble methods are able to achieve higher levels of accuracy and generalization than any individual model could on its own.- Ensemble methods are a powerful tool in the machine learning toolkit, allowing us to harness the power of multiple models to achieve better performance and more robust predictions. By combining the strengths of different models, ensemble methods can help us to overcome the limitations of any individual model and improve the overall accuracy and reliability of our machine learning systems.
Similar Posts
Smart machines are revolutionizing healthcare
In healthcare, the impact of smart machines is profound. These machines are changing the way we approach patient care, diagnosi...
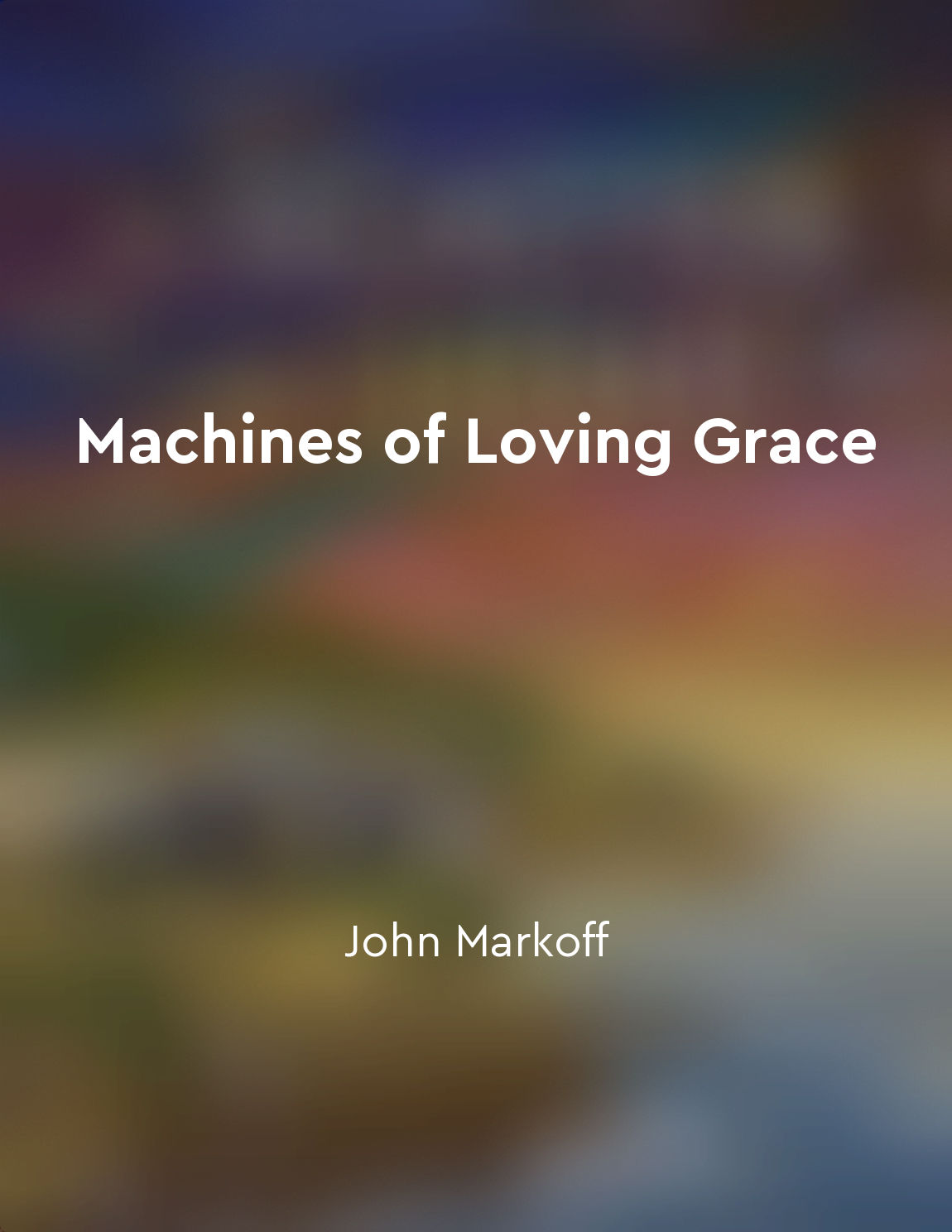
Humans are collaborating with machines
John Markoff explores the intricate relationship between humans and machines in his book "Machines of Loving Grace." The concep...
Programming skills are necessary for data manipulation
To effectively manipulate data, one must possess programming skills. This is because data manipulation involves tasks such as c...
Understand the importance of testing and debugging in Python
Testing and debugging are crucial aspects of programming in Python. When you write code, it's important to test it thoroughly t...
Heat flows from hot to cold bodies
In the study of thermodynamics, a fundamental principle is that heat naturally flows from hot bodies to cold bodies. This princ...
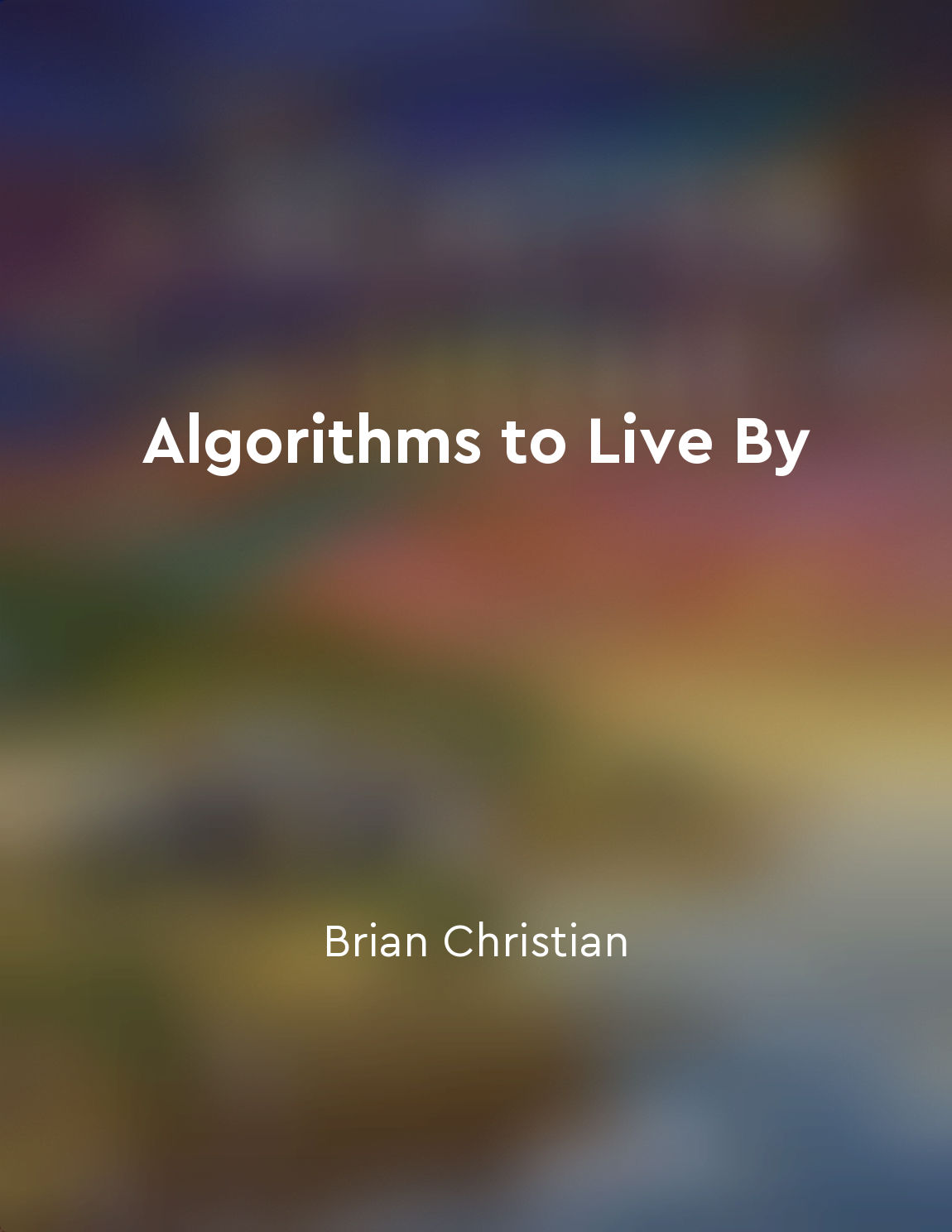
Game theory can help us understand strategic interactions between rational decisionmakers
Game theory offers a framework for understanding how rational decision-makers interact strategically. It provides a way to anal...
Classification models are used to categorize data into classes
Classification models are used to categorize data into classes based on their features. These models are essential in data scie...
Feature selection improves the efficiency of algorithms
Feature selection is a crucial step in machine learning that can significantly improve the efficiency of algorithms. By selecti...