Audio available in app
Ensemble methods combine multiple models to enhance prediction accuracy from "summary" of Introduction to Machine Learning with Python by Andreas C. Müller,Sarah Guido
Ensemble methods are techniques that combine multiple models to enhance prediction accuracy. They can be used in a variety of machine learning tasks, such as classification and regression. By aggregating the predictions of multiple models, ensemble methods are able to produce more reliable and accurate results than any single model on its own. One common type of ensemble method is the bagging meta-estimator, which works by training multiple instances of the same base estimator on different subsets of the training data. These individual models are then combined by averaging their predictions, resulting in a final prediction that is more robust and less prone to overfitting. Bagging can be particularly effective when the base estimator is unstable or has high variance. Another popular ensemble method is the random forest, which is a specific implementation of bagging using decision trees as the base estimator. Random forests are able to reduce the variance of individual decision trees by introducing randomness into the training process, such as by using random subsets of features at each split. This helps to decorrelate the individual trees and improve the overall performance of the ensemble. Boosting is another type of ensemble method that works by training a sequence of models, each of which focuses on the mistakes made by the previous models. By iteratively adjusting the weights of training instances based on their prediction errors, boosting is able to learn from the weaknesses of earlier models and improve the overall accuracy of the ensemble. Popular boosting algorithms include AdaBoost and Gradient Boosting. Ensemble methods can also be combined with other techniques, such as stacking, which involves training a meta-model on the predictions of multiple base models. This allows the meta-model to learn how to best combine the individual predictions to produce a final output with improved accuracy. Stacking is a powerful technique that can further enhance the performance of ensemble methods and is commonly used in machine learning competitions.- Ensemble methods are a powerful tool in the machine learning toolkit, capable of significantly improving prediction accuracy by combining multiple models in a clever and strategic way. By leveraging the diversity of individual models and learning from their strengths and weaknesses, ensemble methods are able to achieve superior performance compared to any single model on its own.
Similar Posts
Python is used in automation and scripting tasks
When you hear about Python being used in automation and scripting tasks, it's essentially referring to the fact that Python mak...
Making precise forecasts can lead to greater accuracy
Being able to make precise forecasts can indeed lead to greater accuracy in predicting future events. When forecasters are able...
Rightcensored data poses its own challenges
Right-censored data poses its own challenges. When we observe values below a certain threshold, we know the exact value. Howeve...
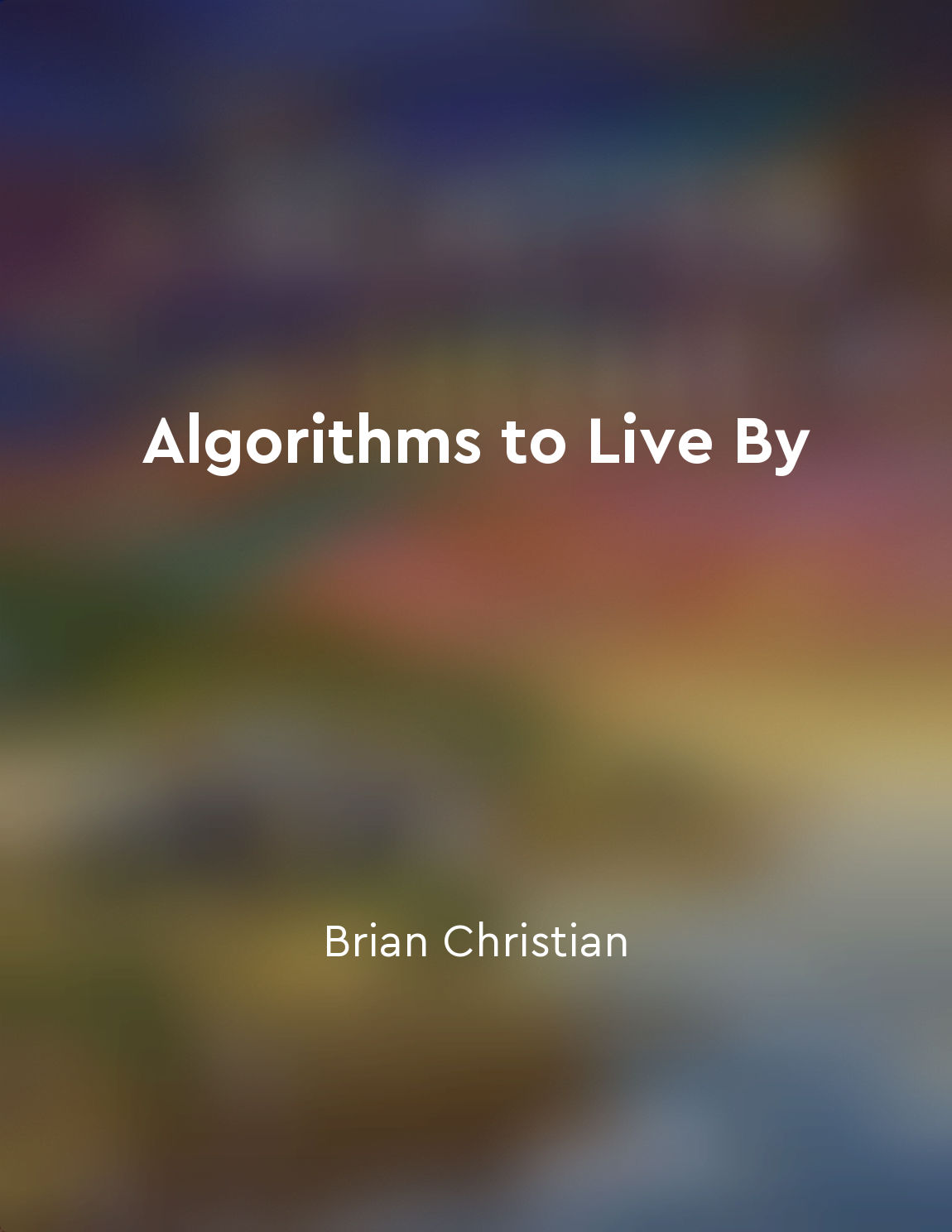
The "randomness" factor plays a role when we lack information to make a decision
When faced with a decision, our choices are often influenced by the information we have available. In some cases, we may lack c...
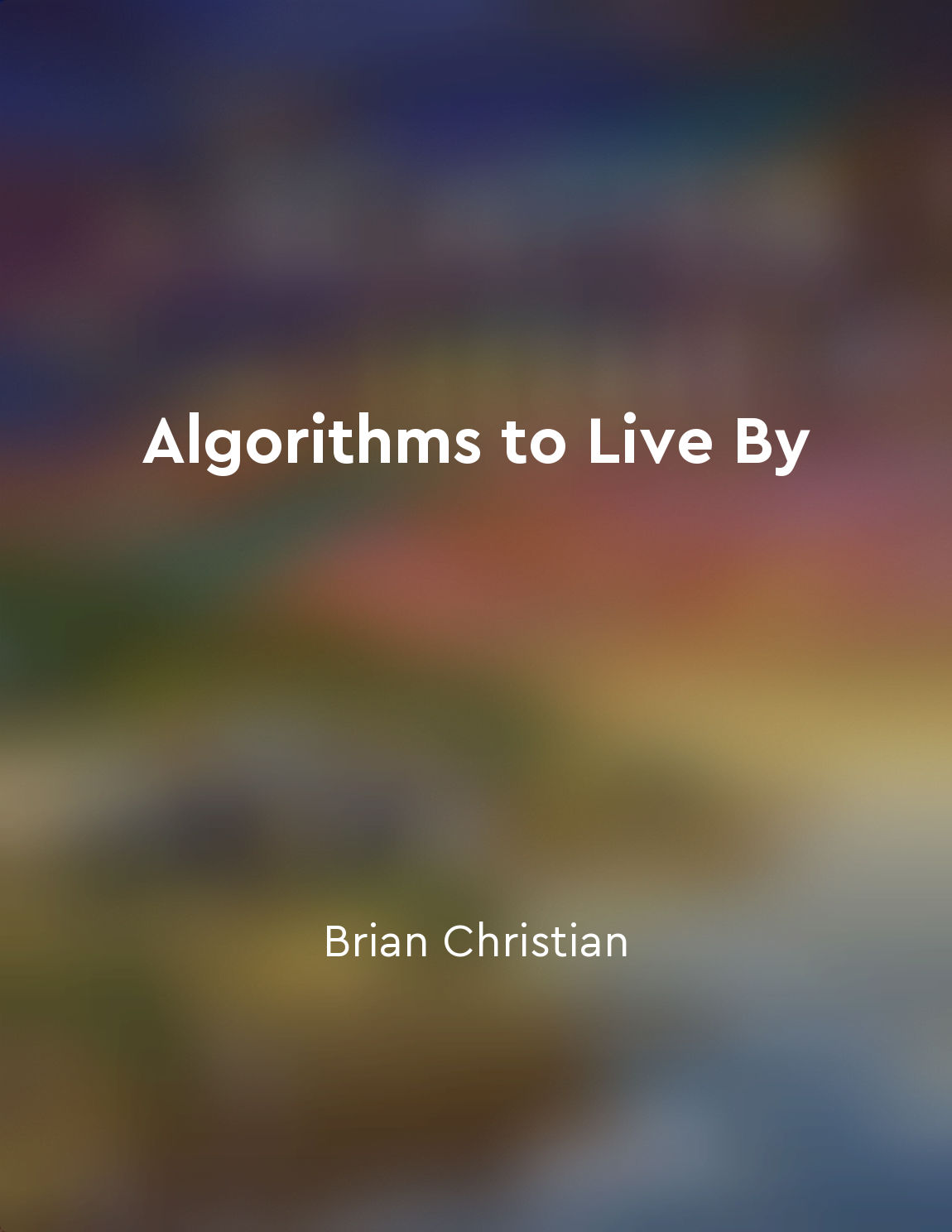
The explore/exploit dilemma teaches us when to try something new and when to stick with what works
The explore/exploit dilemma is a fundamental concept that can guide our decision-making in various aspects of life. It forces u...