Resampling methods can assess the robustness of results from "summary" of Statistics for Censored Environmental Data Using Minitab and R by Dennis R. Helsel
Resampling methods provide a way to test the stability of results by repeatedly sampling from the data. This technique involves generating multiple datasets by randomly selecting observations from the original dataset. By analyzing these resampled datasets, researchers can assess the variability and robustness of their results. One common resampling method is bootstrapping, which involves creating numerous bootstrap samples by sampling with replacement from the original dataset. This approach allows researchers to estimate the variability of a statistic without making assumptions about the underlying distribution of the data. By repeatedly resampling the data, researchers can examine the range of possible outcomes and evaluate the stability of their results. Another resampling method is cross-validation, which involves splitting the data into training and testing sets multiple times. By fitting models to the training data and then testing them on the holdout data, researchers can assess the generalizability of their models. Cross-validation helps to identify overfitting and evaluate the robustness of statistical models. Resampling methods can also be used to assess the sensitivity of results to different assumptions or modeling choices. By varying parameters or assumptions in the resampling process, researchers can explore how different scenarios affect their results. This sensitivity analysis can help researchers understand the limitations of their analyses and make more informed decisions.- Resampling methods provide a powerful tool for assessing the robustness of results in statistical analyses. By generating multiple datasets and exploring different scenarios, researchers can gain insight into the variability and stability of their results. This approach can help researchers make more reliable conclusions and improve the quality of their statistical analyses.
Similar Posts
Natural language processing enables computers to understand and generate human language
Natural language processing (NLP) is an important field in data science that deals with the interaction between computers and h...
Hypothesis testing helps make informed decisions
In hypothesis testing, we start with a null hypothesis that we want to either reject or fail to reject. The null hypothesis typ...
Residual analysis assesses whether the assumptions of the regression model are met
Residual analysis plays a crucial role in determining the validity of a regression model by examining whether the assumptions u...
Understanding censoring mechanisms is key for accurate results
To ensure accurate results from statistical analysis of censored environmental data, it is essential to have a thorough underst...
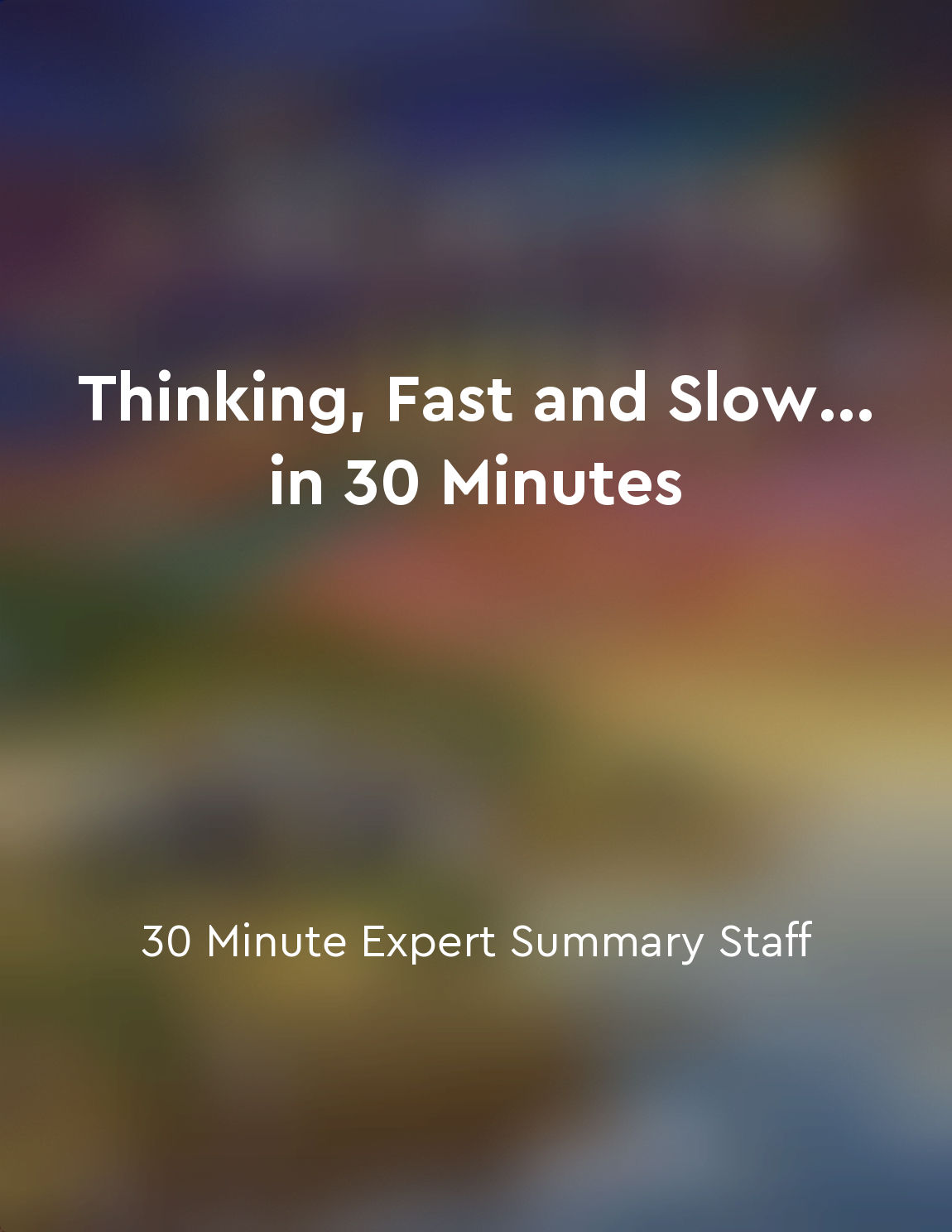
Heuristics provide mental shortcuts
Heuristics, as described by Kahneman, are mental shortcuts that help individuals make decisions quickly and efficiently. These ...