Audio available in app
Challenges exist in predictive modeling from "summary" of Predictive Analytics by Eric Siegel
Predictive modeling is an incredibly powerful tool, allowing organizations to make informed decisions and predict future outcomes based on historical data. However, it is important to recognize that challenges exist in this process that can impact the accuracy and effectiveness of predictive models. One of the main challenges in predictive modeling is the quality of the data being used. Data can be incomplete, inaccurate, or biased, which can lead to misleading results. It is essential to carefully clean and preprocess data before building a predictive model to ensure its reliability and validity. Another challenge is the complexity of the models themselves. As models become more sophisticated, they can become harder to interpret and explain. This lack of transparency can lead to difficulties in understanding how predictions are being made and can make it challenging to gain buy-in from stakeholders. Furthermore, overfitting is a common issue in predictive modeling. This occurs when a model is overly complex and fits too closely to the training data, leading to poor performance on new data. It is crucial to strike a balance between model complexity and generalizability to avoid overfitting. In addition, the choice of algorithms used in predictive modeling can also pose a challenge. Different algorithms have different strengths and weaknesses, and selecting the most appropriate algorithm for a specific problem can be difficult. It is essential to experiment with multiple algorithms and tune their parameters to find the best fit for the data. Finally, ethical considerations must be taken into account in predictive modeling. Biases can be inadvertently introduced into models, leading to unfair or discriminatory outcomes. It is essential to regularly assess and mitigate biases in predictive models to ensure fair and equitable results.- While predictive modeling offers significant benefits, it is not without its challenges. By addressing issues related to data quality, model complexity, overfitting, algorithm selection, and ethical considerations, organizations can build more reliable and effective predictive models.
Similar Posts
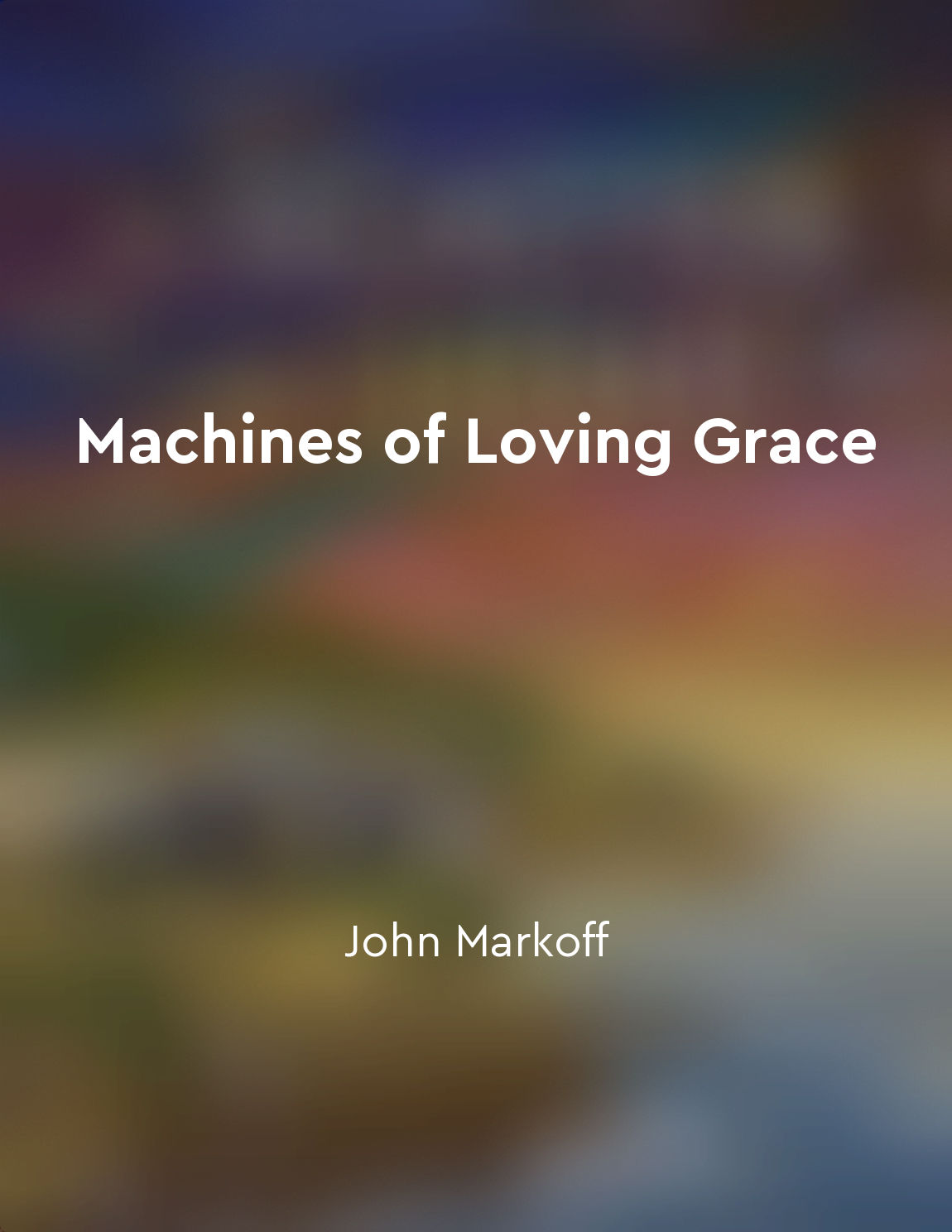
The future of AI is unpredictable
As we gaze into the future of artificial intelligence, one thing becomes abundantly clear: unpredictability reigns supreme. The...
Supervised learning involves training a model on labeled data
Supervised learning is a type of machine learning where we have a specific outcome we want to predict or classify. In this sett...
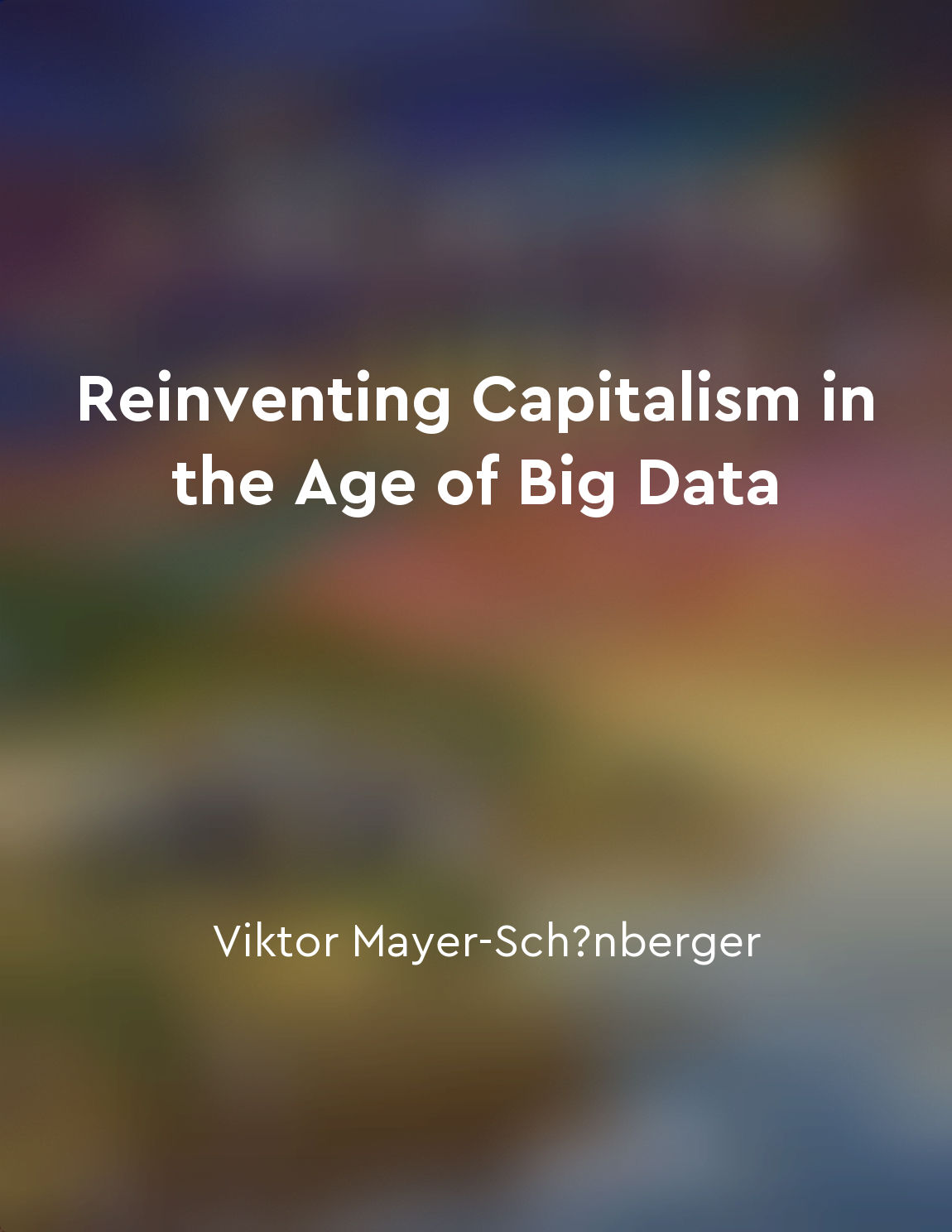
Data ethics should be at the forefront of business decisions
In our modern age of big data, the ethical implications of how companies collect, use, and protect data have become increasingl...
Modules help organize code
When writing a large program, it's important to keep your code organized. One way to do this is by using modules. Modules are f...