Audio available in app
Evaluating model performance requires various metrics from "summary" of Machine Learning For Dummies by John Paul Mueller,Luca Massaron
When you create a machine learning model, you need to know how well it performs in order to make improvements. Evaluating the performance of a model requires looking at various metrics. These metrics help you understand how accurate your model is and where it can be improved. One common metric for evaluating classification models is accuracy. Accuracy tells you the percentage of correctly classified instances out of the total instances. While accuracy is a good starting point, it may not tell the whole story. For example, if you have imbalanced classes, accuracy may not be a reliable metric. Precision and recall are two other important metrics for evaluating classification models. Precision tells you the percentage of correctly classified positive instances out of all instances classified as positive. Recall, on the other hand, tells you the percentage of correctly classified positive instances out of all actual positive instances. These two metrics can give you a better understanding of how well your model is performing, especially when dealing with imbalanced classes. Another important metric for evaluating classification models is the F1 score, which is the harmonic mean of precision and recall. The F1 score gives you a balanced view of both precision and recall and can be a good overall metric for model performance. For regression models, one common metric is the mean squared error (MSE). MSE measures the average squared difference between the predicted values and the actual values. A lower MSE indicates a better-performing model. In addition to these metrics, there are many others you can use to evaluate model performance, depending on the specific problem you are trying to solve. By looking at a combination of metrics, you can get a more comprehensive understanding of how well your model is performing and where it can be improved.Similar Posts
Outlier detection is crucial for data quality
The identification of outliers in a dataset is a critical step in ensuring the quality and reliability of the data. Outliers ar...
Seek feedback and iterate
At Google, we have a simple philosophy when it comes to improving our work: seek feedback and iterate. This concept is at the c...
Adapt to changing market conditions
To succeed in business, it is crucial to be able to adjust to the ever-changing landscape of the market. Market conditions are ...
Deep learning involves multiple layers of neural networks for complex tasks
Deep learning is a subfield of machine learning that is concerned with algorithms inspired by the structure and function of the...
Engage with customers through storytelling
Storytelling is a powerful tool that businesses can use to connect with their customers on a deeper level. By sharing stories t...
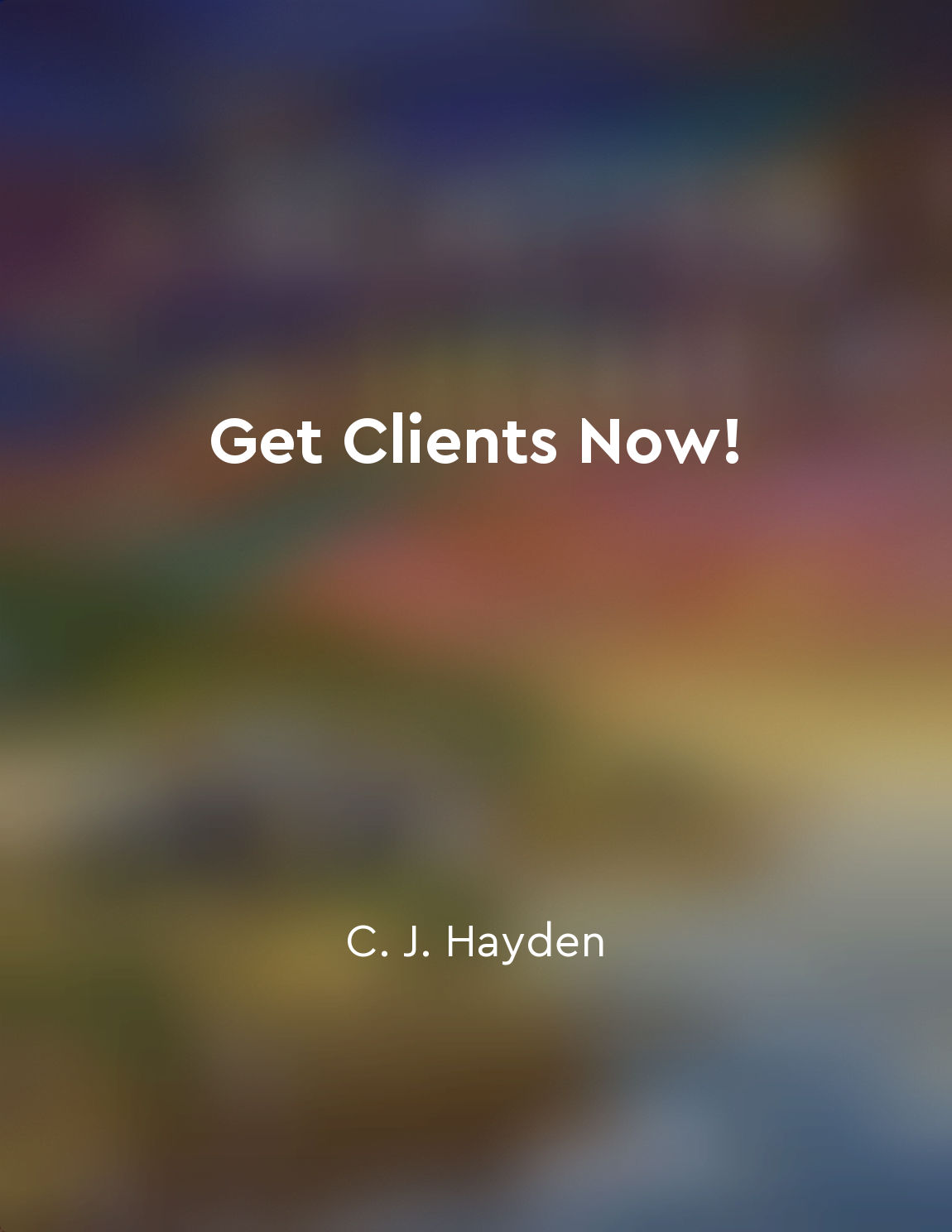
Network with others to expand your client base
One of the most effective ways to grow your client base is by connecting and collaborating with others in your industry. By net...
Neural networks are a powerful tool for modeling complex relationships in data
Neural networks have gained popularity in data science due to their ability to capture complex relationships within data. These...
Big data refers to large datasets that require special tools
Big data is all about dealing with massive amounts of data that traditional data processing tools struggle to handle. The term ...
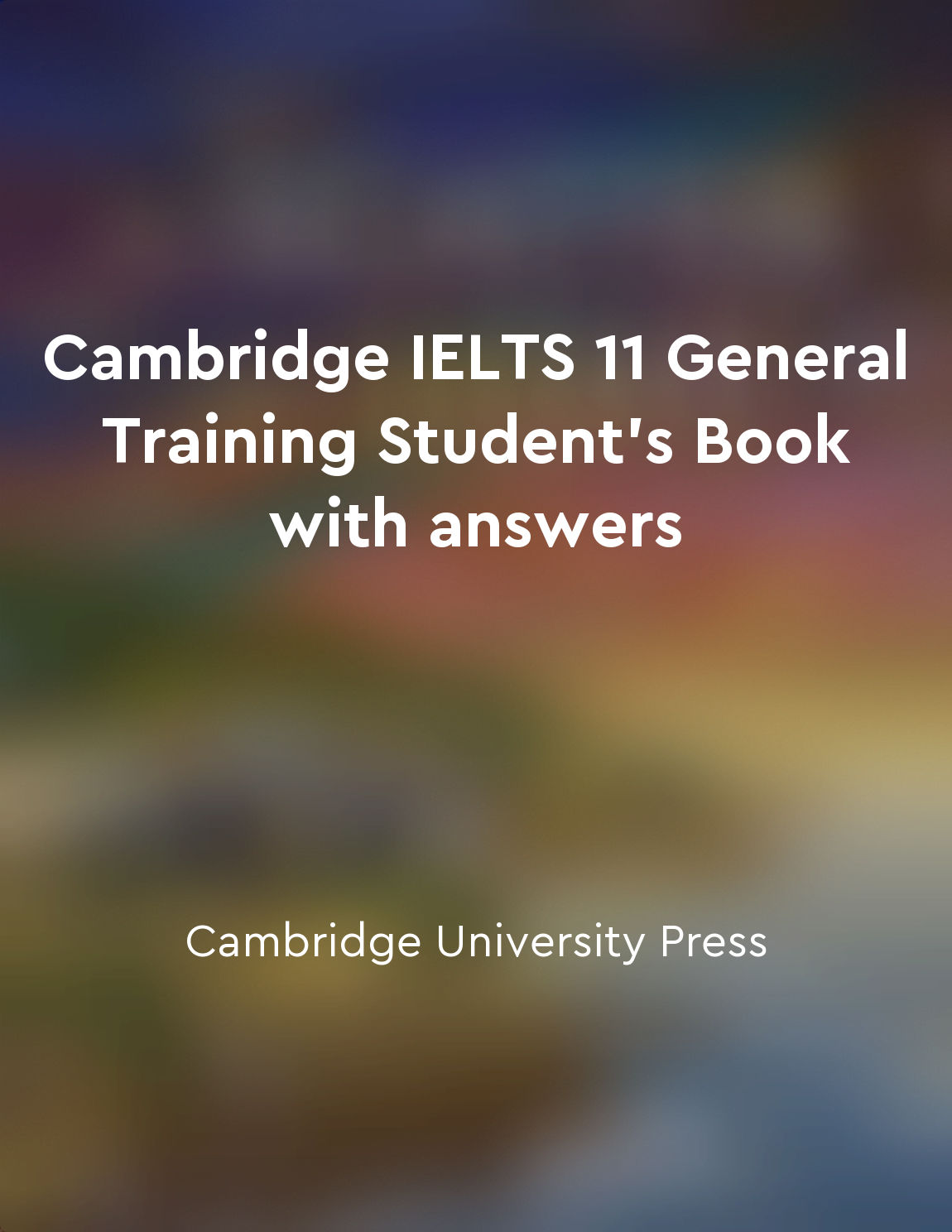
Key idea 6: Practicing for the speaking test with sample questions
To help you prepare for the speaking test, it is essential to practice with sample questions. By practicing with these question...
Celebrate achievements and milestones to recognize and reward progress and success
Acknowledging and commemorating achievements and milestones is a crucial aspect of fostering a positive and productive work env...