Residual analysis assesses whether the assumptions of the regression model are met from "summary" of Introduction to Econometrics by Christopher Dougherty
Residual analysis plays a crucial role in determining the validity of a regression model by examining whether the assumptions underlying the model hold true. These assumptions are essential for ensuring the reliability of the model's results and the accuracy of its predictions. Residuals are essentially the differences between the observed values and the values predicted by the regression model. By analyzing these residuals, we can gain valuable insights into the model's performance and identify any potential issues or violations of the underlying assumptions. One key assumption of regression analysis is that the residuals should be randomly distributed around zero. This implies that there should be no systematic patterns or trends in the residuals, as any such patterns could indicate that the model is missing important variables or relationships. Residual analysis helps us detect such patterns by plotting the residuals against the predicted values or other relevant variables. If we observe a clear pattern in these plots, it suggests that the model may be misspecified or that the underlying assumptions are not being met. Another important assumption is that the residuals should have constant variance, also known as homoscedasticity. This means that the spread of the residuals should remain consistent across different levels of the independent variables. Residual analysis helps us check for homoscedasticity by examining plots of the residuals against the predicted values or other relevant variables. If we notice that the spread of the residuals is not uniform or that it varies systematically, it indicates a violation of the homoscedasticity assumption. Additionally, residual analysis can help us detect outliers or influential observations that may have a disproportionately large impact on the regression results. Outliers are data points that deviate significantly from the overall pattern of the data, while influential observations are data points that have a strong influence on the estimated regression coefficients. By identifying and examining these observations, we can assess their impact on the model and determine whether they should be excluded or treated differently in the analysis. In summary, residual analysis is a critical tool for evaluating the assumptions of a regression model and ensuring the validity of its results. By carefully examining the residuals and their properties, we can identify potential issues such as misspecification, nonlinearity, heteroscedasticity, outliers, and influential observations. Addressing these issues is essential for building a reliable and accurate regression model that can provide meaningful insights into the relationships between the variables under study.Similar Posts
Keeping up with advancements in machine learning ensures competitiveness
Staying current with the latest developments in machine learning is essential for maintaining a competitive edge in today's fas...
Machine learning algorithms help in predicting outcomes
Machine learning algorithms are essential tools for data scientists because they can help predict outcomes based on data patter...
People seek out information that confirms their beliefs
The concept of seeking out information that confirms one's existing beliefs is a common tendency among individuals. When people...
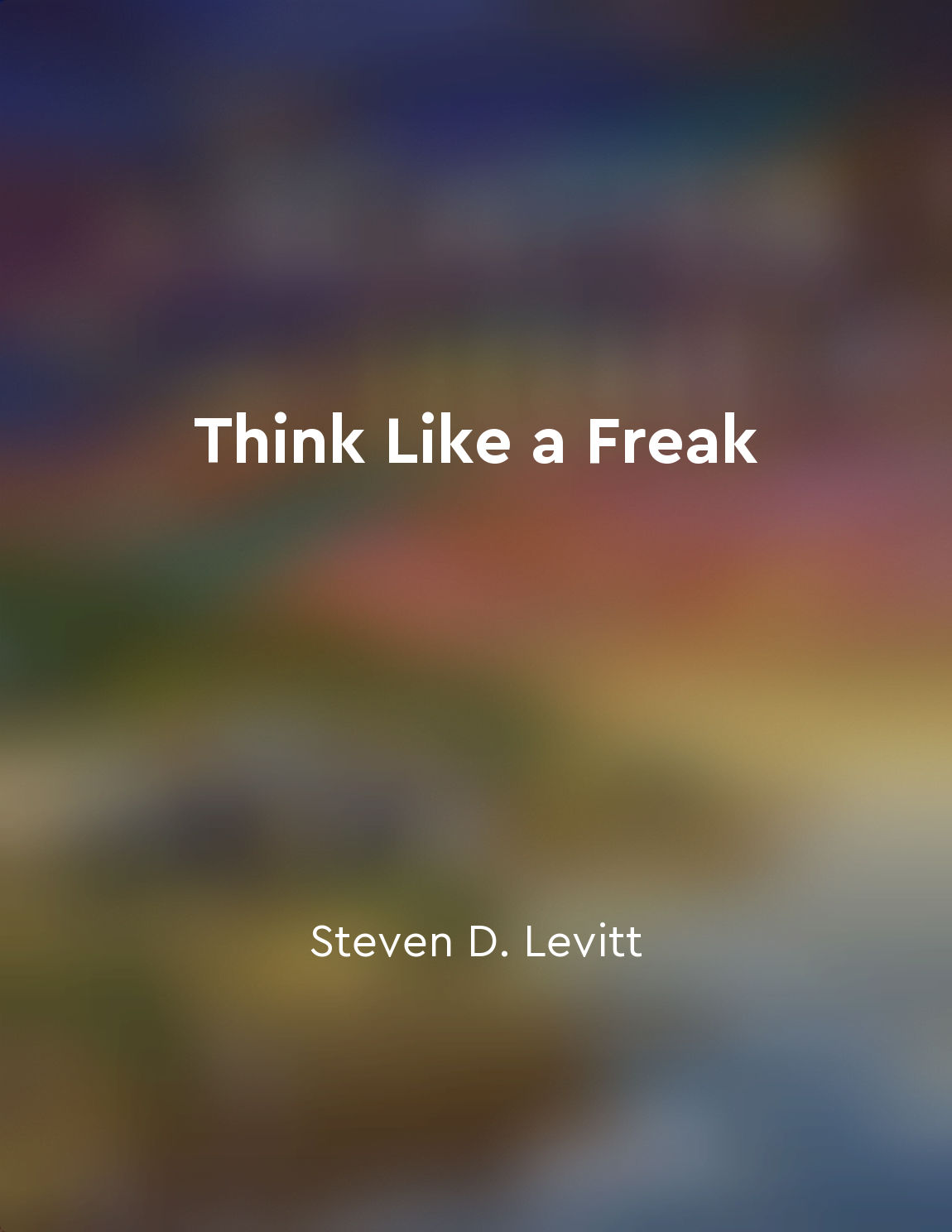
Learn from successes and failures
The idea of learning from successes and failures is not a new concept. Yet, it is one that is often overlooked or not fully uti...
Test assumptions to uncover hidden insights
When faced with a complex problem, it is crucial to test assumptions in order to reveal hidden insights that may not be immedia...
Use fundamental analysis to evaluate stocks
Fundamental analysis is a key concept in evaluating stocks for potential investment. This approach involves examining a company...
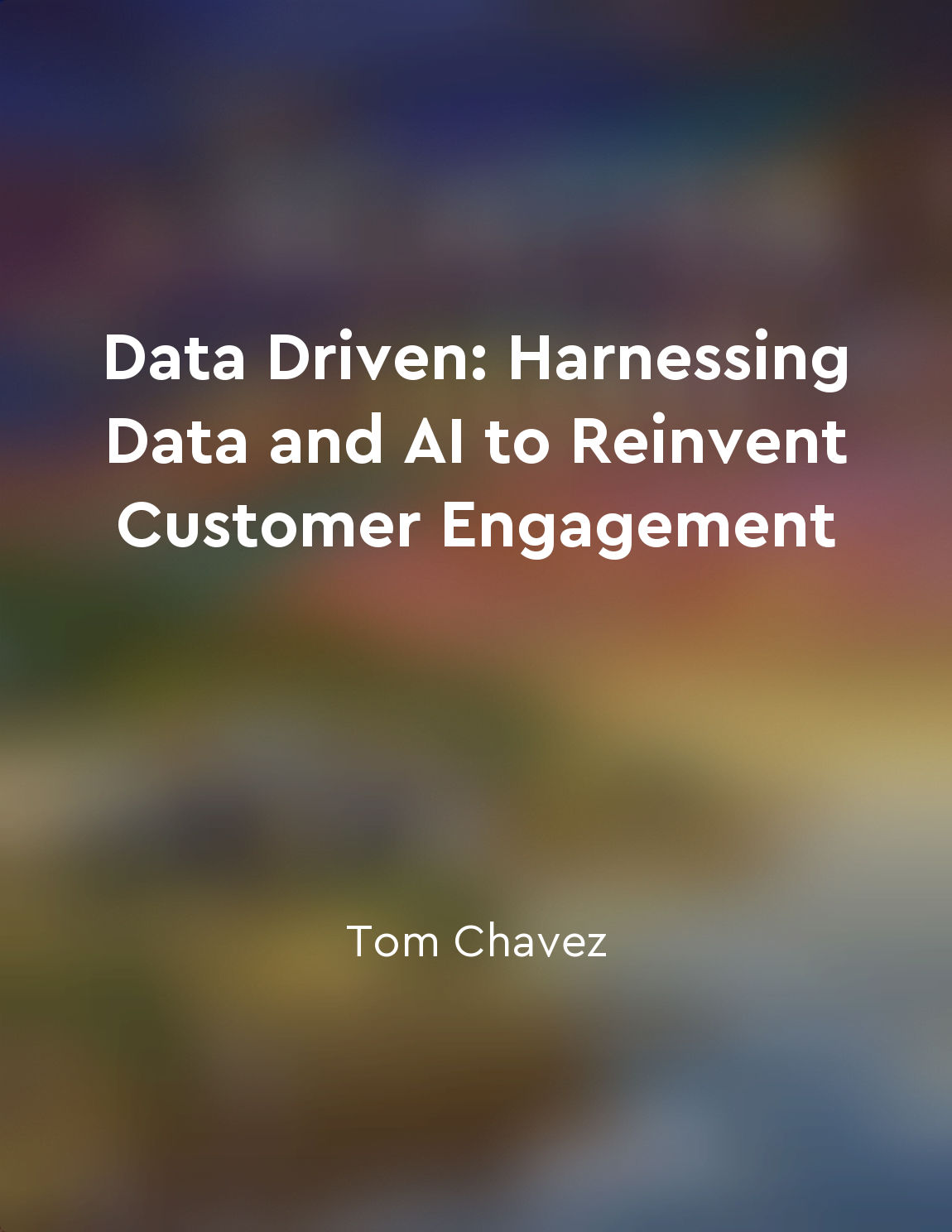
Datadriven decision-making leads to success
The power of data in driving decision-making cannot be underestimated. Data is not just numbers or statistics; it is a goldmine...
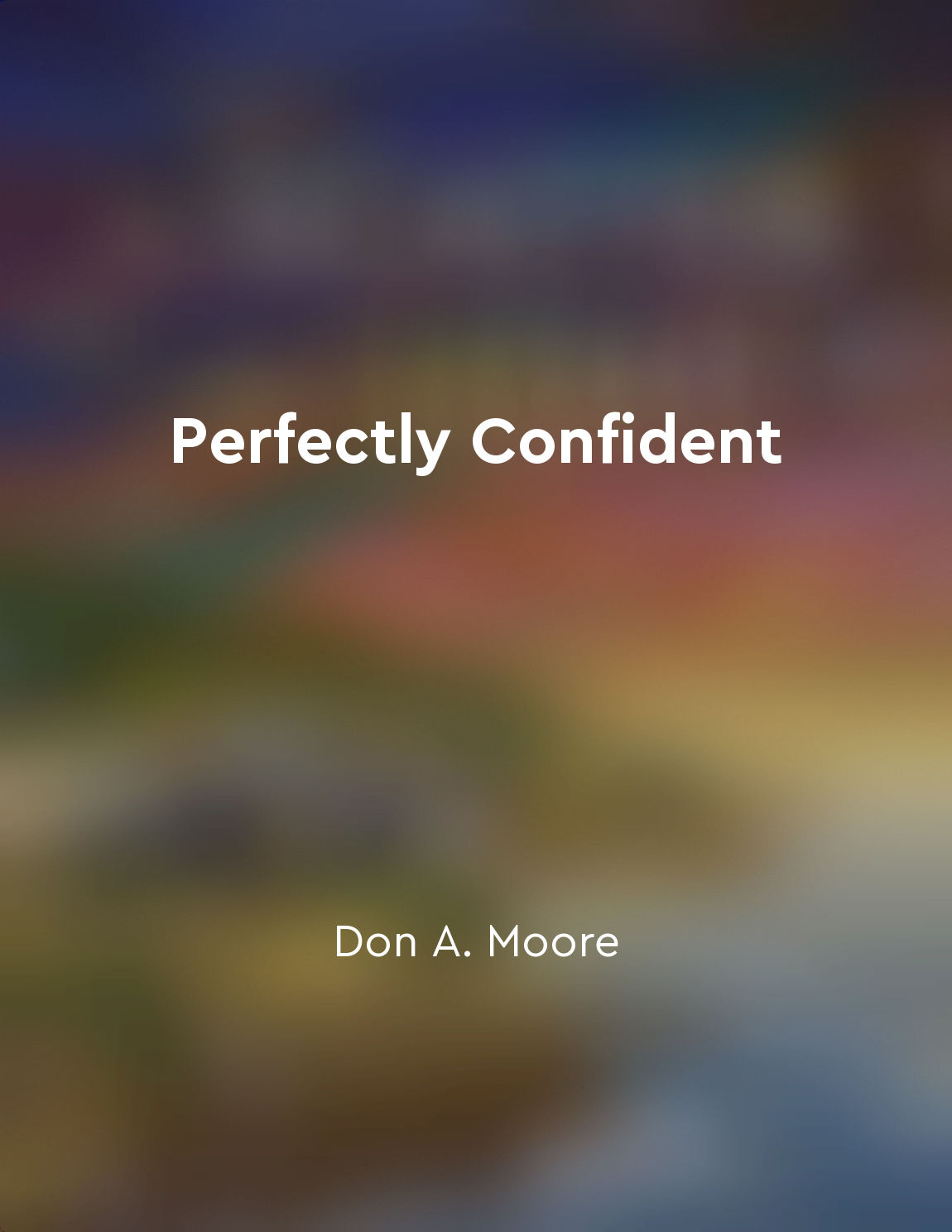
Understand the limits of your knowledge
It's important to acknowledge that our knowledge has boundaries. We can't possibly know everything about a particular subject o...
Setting goals provides direction for problemsolving efforts
Goals play a crucial role in guiding problem-solving efforts. When individuals set clear and specific goals, they provide thems...
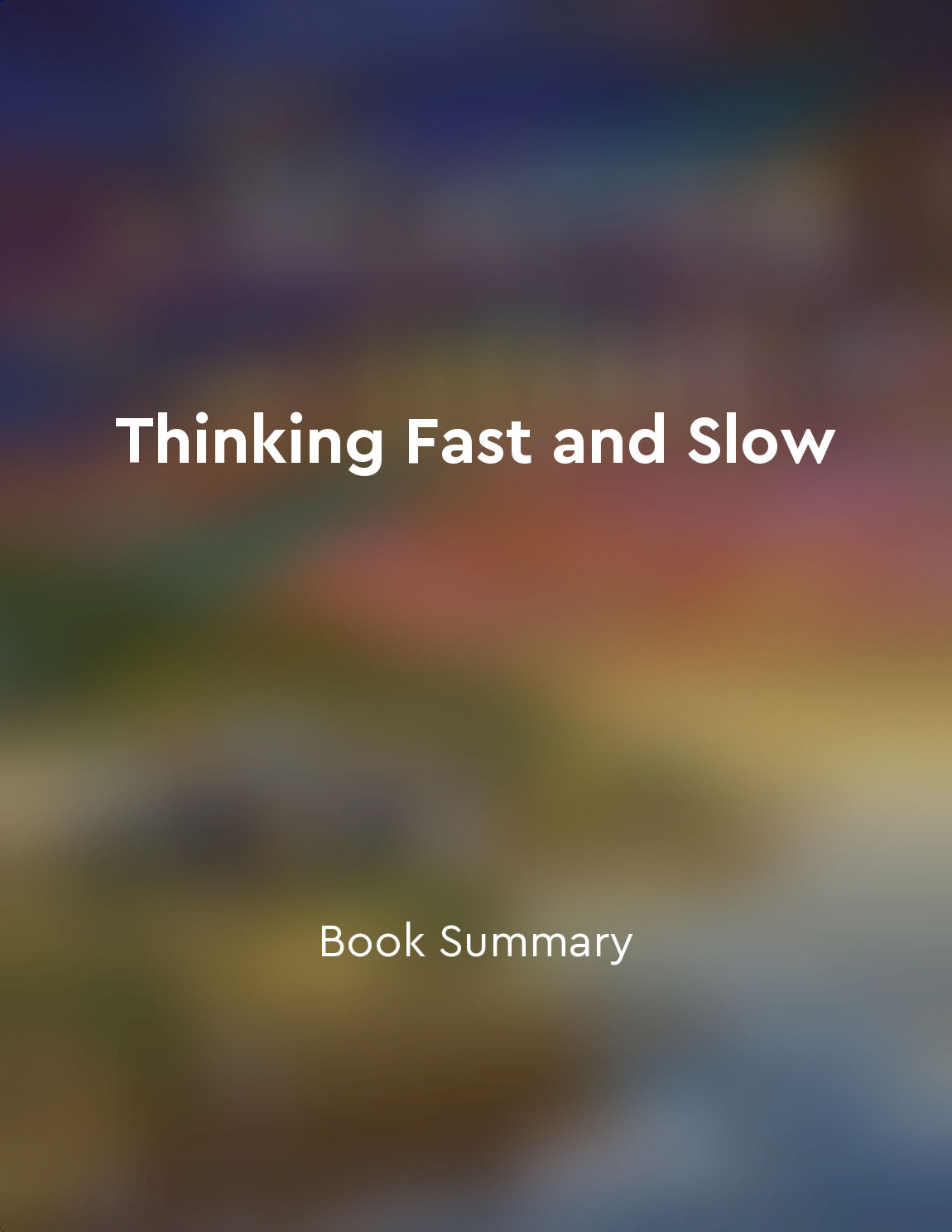
System 2 requires conscious effort and attention
System 2, as I have explained, is the slower, more deliberate mode of thinking that requires conscious effort and attention. Wh...