Audio available in app
Ensemble methods combine multiple models to improve prediction accuracy from "summary" of Data Science for Business by Foster Provost,Tom Fawcett
Ensemble methods refer to the practice of combining multiple models to improve prediction accuracy. Instead of relying on a single model to make predictions, ensemble methods leverage the strength of a diverse set of models to arrive at a more accurate prediction. Each model in the ensemble may have its own strengths and weaknesses, which can be mitigated by combining them with other models that complement their performance. There are several ways to create an ensemble of models. One common approach is to train multiple models on different subsets of the data and then combine their predictions using a technique such as averaging or a voting mechanism. This allows the ensemble to benefit from the diversity of the individual models, as errors made by one model may be offset by the correct predictions of another. Another popular method of creating an ensemble is through the use of bagging or boosting. Bagging involves training multiple models on bootstrap samples of the data and then combining their predictions. This helps to reduce variance and improve overall prediction accuracy. Boosting, on the other hand, involves training models sequentially, with each new model focusing on the errors made by the previous models. This iterative process can lead to significant improvements in prediction accuracy. Ensemble methods have been shown to be highly effective in practice, often outperforming individual models in terms of prediction accuracy. By leveraging the strengths of multiple models and mitigating their weaknesses, ensembles can provide more robust and reliable predictions. This approach is particularly useful in situations where the data is noisy or complex, as the diversity of the ensemble can help to capture different aspects of the underlying patterns in the data.- Ensemble methods offer a powerful tool for improving prediction accuracy in data science applications. By combining multiple models in a systematic way, ensembles can provide more accurate and reliable predictions than individual models alone. This approach has been successfully applied in a wide range of domains, demonstrating the effectiveness and versatility of ensemble methods in practice.
Similar Posts
Natural language processing enables computers to understand and generate human language
Natural language processing (NLP) is an important field in data science that deals with the interaction between computers and h...
Unstructured data includes text, images, and videos
Unstructured data is a term used to describe any type of data that does not fit neatly into a structured format. This includes ...
Python is a versatile language used in various industries
Python is a versatile language that is widely used across various industries due to its simplicity and ease of learning. Its cl...
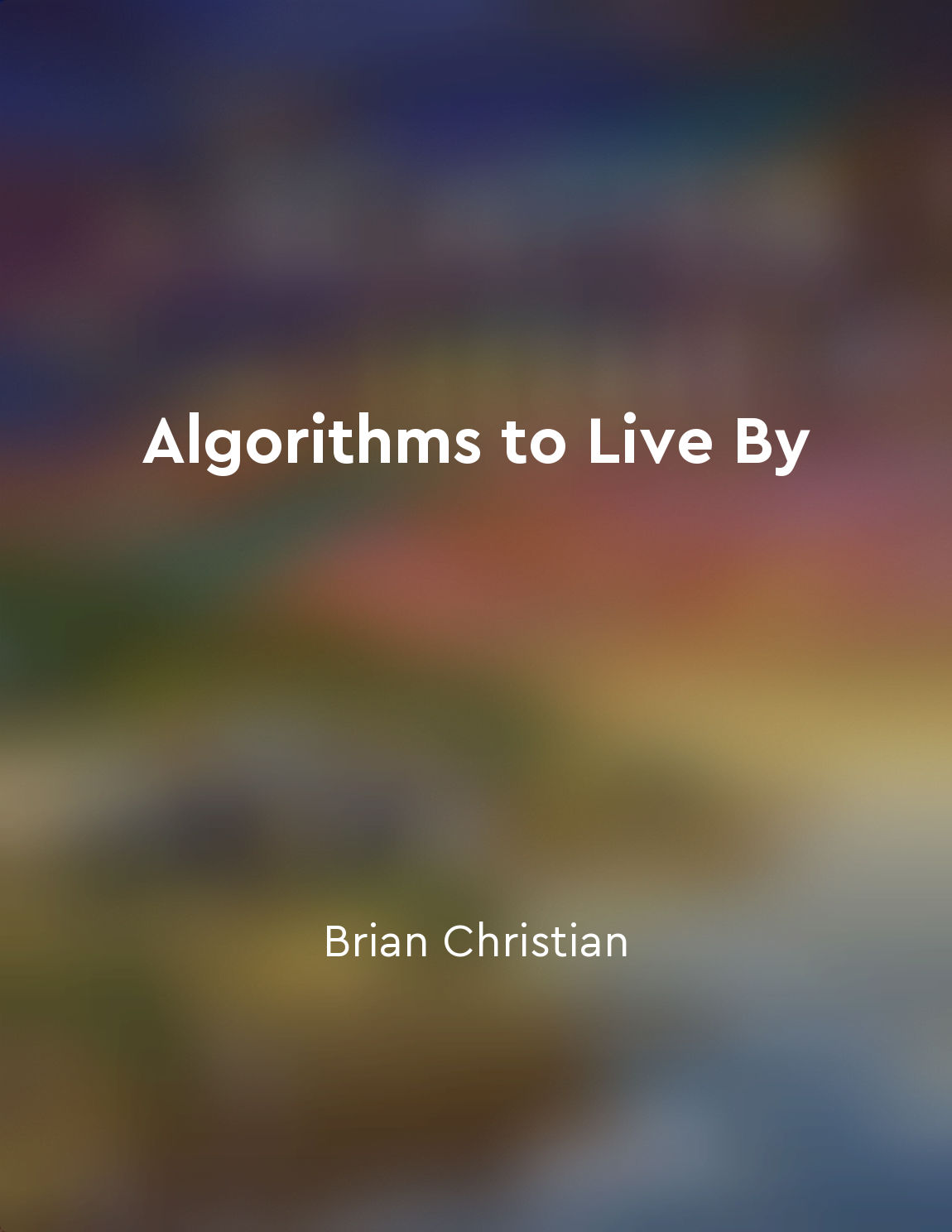
Randomness can help us make decisions when we lack information
Imagine you're faced with a difficult decision, but you lack crucial information to make an informed choice. What do you do in ...
Superforecasters are willing to consider alternative viewpoints
Superforecasters possess a unique ability that sets them apart from others in their field. They are not rigid in their thinking...
Feature selection is important for improving model accuracy
Feature selection is crucial for improving model accuracy. Not all features are equally valuable for prediction, and some may e...