Outlier detection is crucial for data quality from "summary" of Statistics for Censored Environmental Data Using Minitab and R by Dennis R. Helsel
The identification of outliers in a dataset is a critical step in ensuring the quality and reliability of the data. Outliers are data points that deviate significantly from the rest of the data and can have a disproportionate impact on statistical analyses. These data points can arise due to errors in data collection, measurement variability, or true differences in the underlying processes being studied. Failure to detect and address outliers can lead to biased estimates, incorrect conclusions, and reduced statistical power. Outliers can skew the distribution of the data, affect the calculation of summary statistics, and influence the results of hypothesis tests. By identifying and removing outliers, researchers can improve the accuracy and precision of their analyses, leading to more robust and trustworthy results.
There are various methods available for detecting outliers, including graphical techniques, statistical tests, and modeling approaches. Graphical methods, such as boxplots and scatter plots, can provide visual cues to the presence of outliers in the data. Statistical tests, such as Grubbs' test and Dixon's test, can help identify data points that are significantly different from the rest of the dataset.
In some cases, more advanced modeling techniques, such as robust regression or clustering algorithms, may be necessary to detect outliers in complex datasets. Regardless of the method used, the goal of outlier detection is to identify and investigate data points that may be erroneous or misleading, and to ensure that the data used for analysis is accurate, reliable, and representative of the underlying population.Outlier detection is a crucial step in the data analysis process, as it helps to improve the quality of the data and the validity of the conclusions drawn from statistical analyses. By identifying and addressing outliers, researchers can enhance the credibility and usefulness of their findings, leading to more informed decision-making and better understanding of the phenomena being studied.
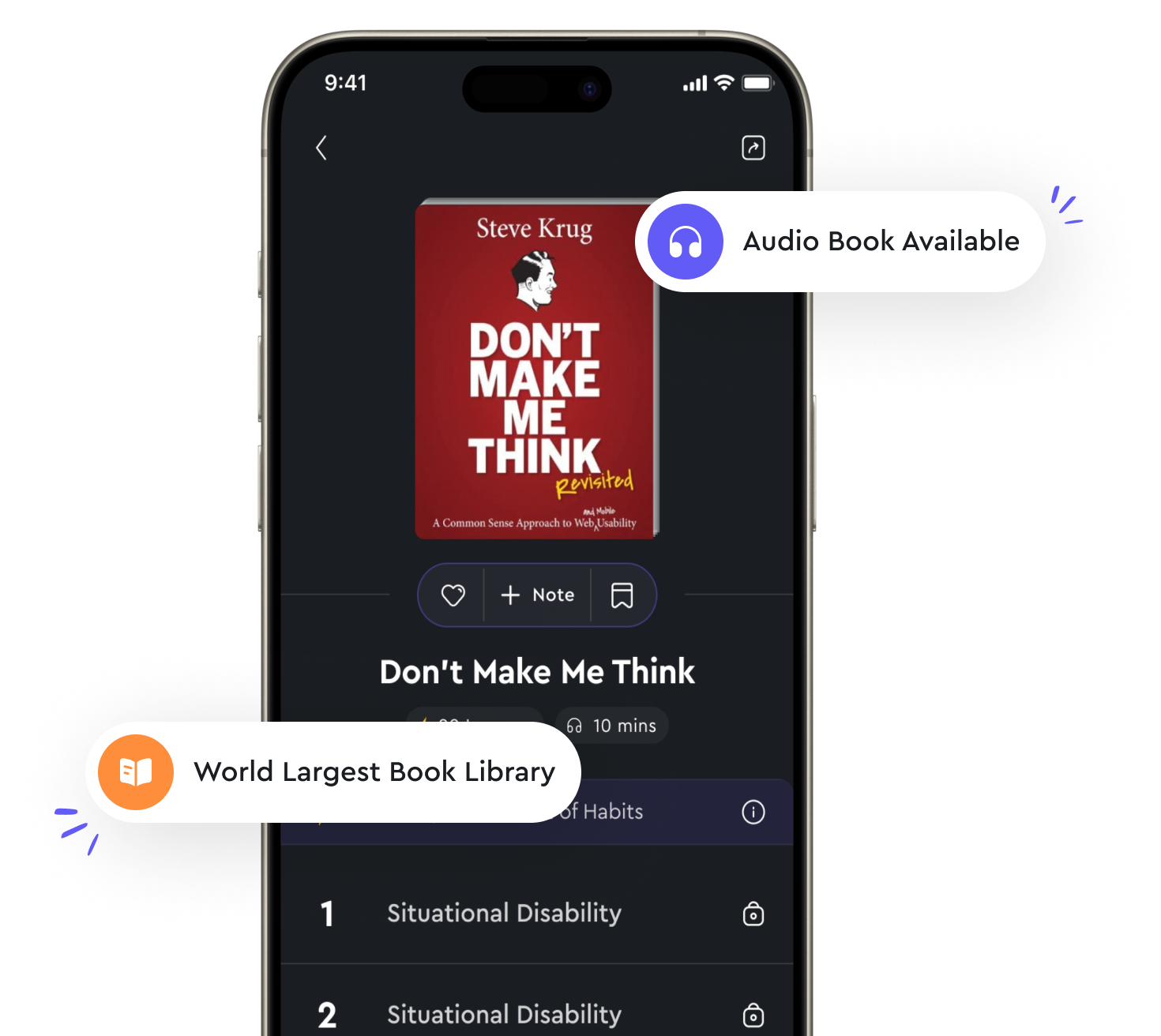
Open in app
The road to your goals is in your pocket! Download the Oter App to continue reading your Microbooks from anywhere, anytime.