Audio available in app
Visualize data with matplotlib and seaborn from "summary" of Python for Data Analysis by Wes McKinney
The process of visualizing data is crucial for understanding patterns and relationships within datasets. Matplotlib is a widely used plotting library in Python that allows users to create a variety of visualizations, such as line plots, scatter plots, bar plots, histograms, and more. Seaborn is a higher-level interface to Matplotlib that simplifies the process of creating attractive and informative visualizations. Matplotlib and Seaborn provide a wide range of customization options for plots, including colors, labels, titles, axes, grids, legends, and more. By leveraging these options, users can tailor their visualizations to effectively communicate insights from the data. For example, adjusting the color palette of a plot can help differentiate between different categories or highlight specific trends. In addition to basic plots, Matplotlib and Seaborn offer advanced visualization techniques, such as faceting, grouping, and aggregation. These techniques enable users to explore complex relationships within multidimensional datasets. For instance, faceting allows users to create multiple plots based on different subsets of the data, while grouping and aggregation help summarize data for clearer interpretation. Furthermore, Matplotlib and Seaborn support interactive plotting, which allows users to explore data dynamically through zooming, panning, and hovering. This interactivity enhances the exploratory data analysis process by enabling users to focus on specific regions of interest or inspect individual data points. By combining interactivity with customization options, users can create engaging and informative visualizations that facilitate data-driven decision-making.- Matplotlib and Seaborn are powerful tools for visualizing data in Python. By leveraging their capabilities, users can create a wide variety of plots that effectively communicate insights from datasets. Whether exploring relationships, identifying trends, or presenting findings, these libraries provide the flexibility and functionality needed to produce compelling visualizations that enhance data analysis and interpretation.
Similar Posts
Volume analysis confirms price movements
Volume analysis plays a critical role in confirming price movements in the market. When the volume of trading activity increase...
Comments document code for others to understand
When writing code, it is crucial to remember that it is not just for the eyes of the person who wrote it. Others will invariabl...
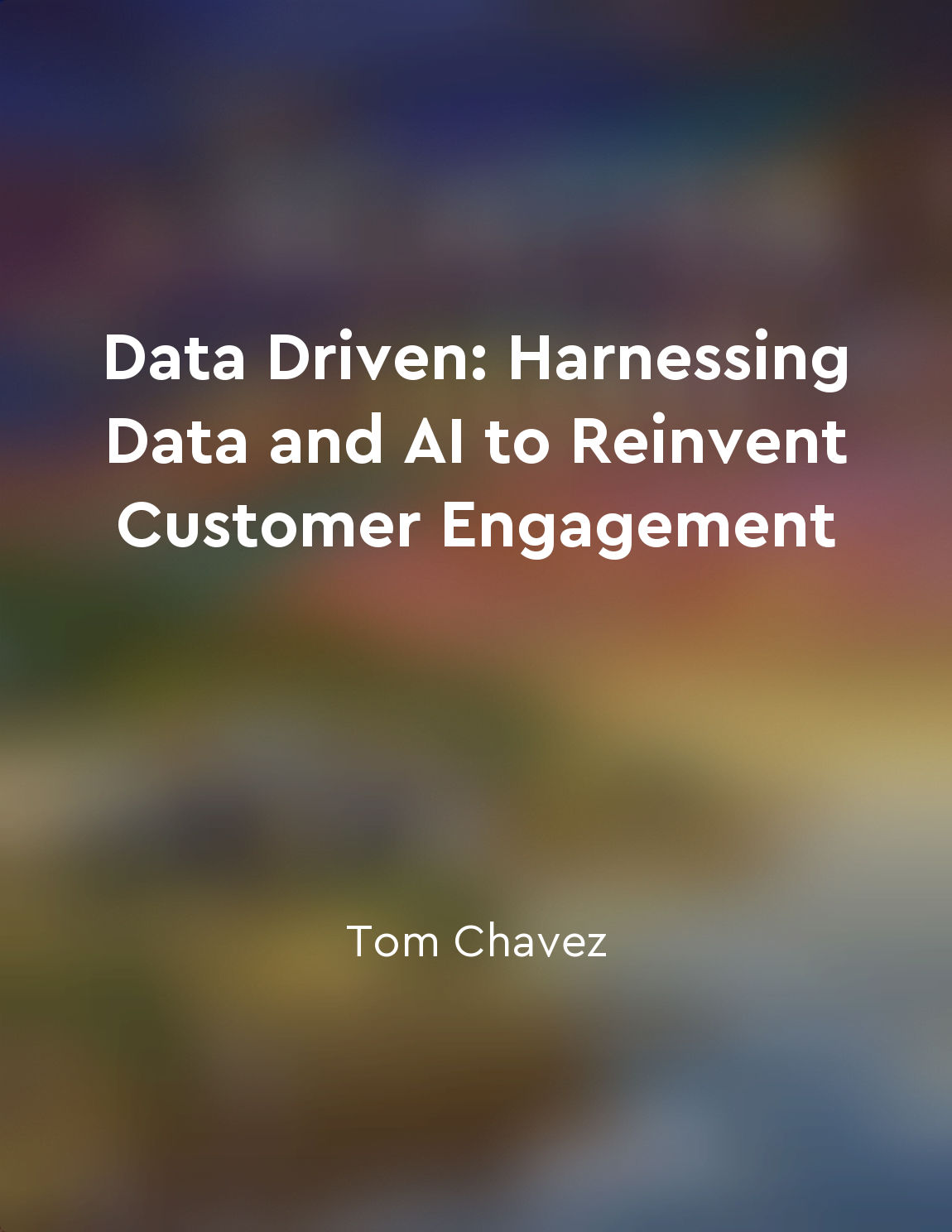
Engagement strategies must evolve
The landscape of customer engagement is constantly changing, driven by advancements in technology, shifts in consumer behavior,...
Engaging in collaborative learning experiences
Collaborative learning experiences are a valuable tool for enhancing your understanding of new concepts. By working with others...
Stakeholder mapping identifies key players in the process
Stakeholder mapping is a process that helps you understand who has a vested interest in a particular experience. By identifying...
Data visualization is a storytelling tool not just a technical endeavor
Data visualization transcends mere technical execution; it serves as a vital medium for storytelling. Effective visualizations ...
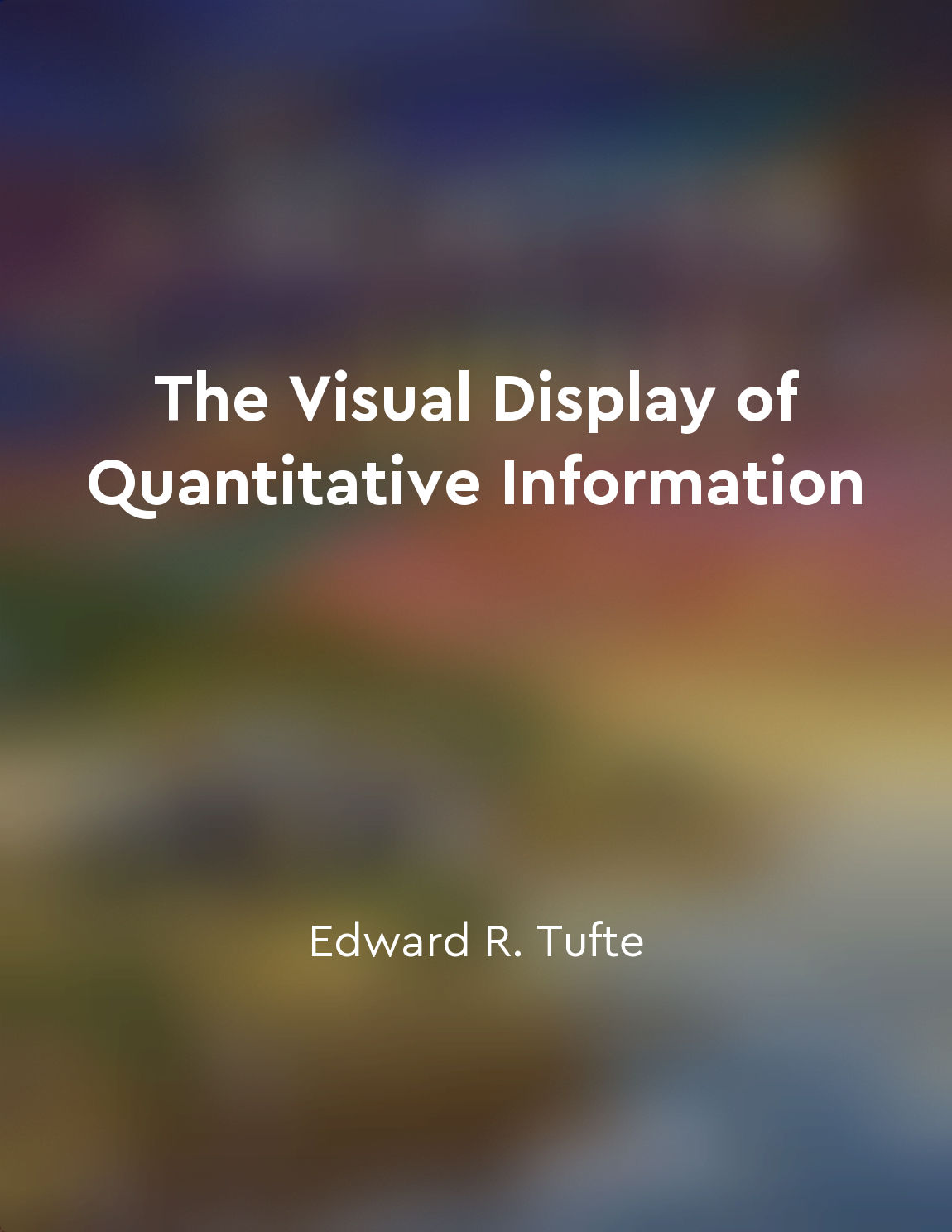
Condense information without sacrificing clarity
To effectively communicate complex information, it is crucial to condense the data without sacrificing clarity. This principle ...
Use modules to organize your Python code
When you start writing Python code, you'll likely find yourself creating more and more functions as your program grows. It can ...
Connect emotionally to create impact
To establish a powerful connection with your audience, it is essential to tap into their emotions. By creating an emotional res...