Audio available in app
Genetic algorithms can optimize solutions through imitation of natural selection from "summary" of Machine Learning by Stephen Marsland
Genetic algorithms are inspired by the process of natural selection, where the fittest individuals in a population are more likely to survive and pass on their genes to the next generation. In the context of optimization problems, genetic algorithms work by evolving a population of potential solutions over multiple generations to find the best solution to a given problem. At the start of the process, a population of potential solutions is generated randomly. Each individual in the population represents a possible solution to the optimization problem. These individuals are then evaluated based on a fitness function that measures how well they perform in solving the problem. The fitter individuals - those that have higher fitness scores - are more likely to be selected for reproduction. During the reproduction phase, pairs of individuals are selected from the population based on their fitness scores. These pairs are then used to create new individuals through a process called crossover, where parts of the solutions from the parent individuals are combined to create new offspring. Mutation is also applied to introduce random changes in the offspring, allowing for exploration of new regions of the solution space. The new offspring then replace some of the individuals in the current population, and the process is repeated for multiple generations. Over time, the population evolves to contain more and more fit individuals, leading to the optimization of the solutions. By imitating the principles of natural selection, genetic algorithms are able to efficiently search through a large space of possible solutions and find the best one for a given problem.- Genetic algorithms provide a powerful optimization technique that can be applied to a wide range of problems in machine learning and artificial intelligence. By mimicking the process of natural selection, genetic algorithms are able to iteratively improve solutions and find optimal results in a computationally efficient manner.
Similar Posts
The necessity of error correction in problemsolving
In order to advance our understanding and solve problems effectively, it is imperative that we embrace and actively engage in e...
Web development uses Python for building web applications
Python is a versatile programming language that is widely used in web development. One of the key reasons for its popularity is...
System resilience is necessary for sustainability
System resilience is necessary for sustainability because it allows a system to adapt and persist through unexpected disturbanc...
Energy is transferred in living organisms through various metabolic pathways
Living organisms rely on various metabolic pathways to transfer energy within their bodies. These pathways involve complex bioc...
Variation within species is essential for evolution
The central tenet of evolutionary theory, as articulated by Charles Darwin and further developed by subsequent scientists, is t...
The impact of AI on the job market is significant
The transformative potential of AI on the job market is nothing short of revolutionary. As technology continues to advance at a...
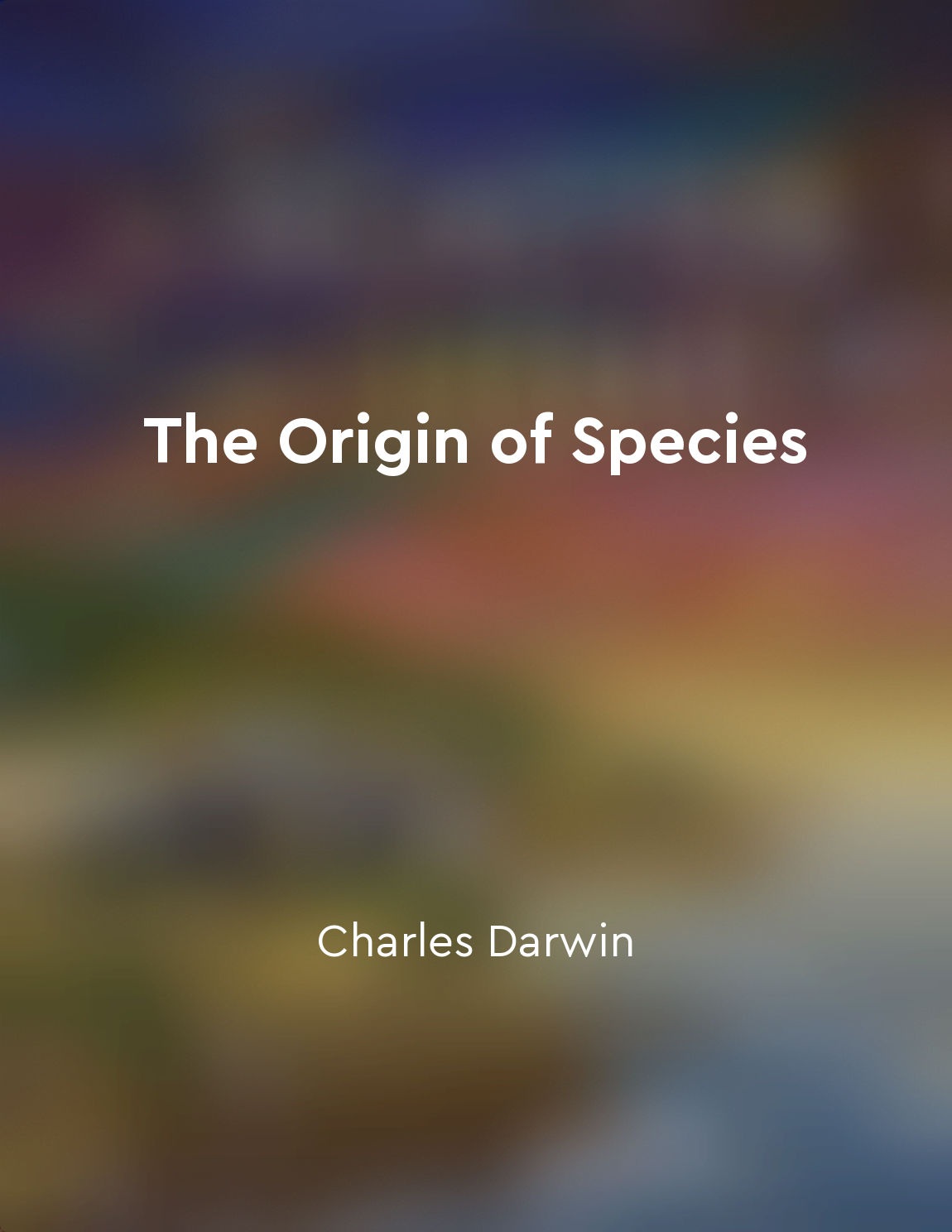
Adaptations enhance survival in changing environments
The ongoing struggle for survival among organisms in nature is a fundamental aspect of the natural world. In this perpetual bat...