Audio available in app
Overfitting must be avoided to ensure model generalization from "summary" of Machine Learning For Dummies by John Paul Mueller,Luca Massaron
Overfitting occurs when a model learns the training data too well, to the point that it starts to memorize the data rather than generalize from it. In other words, the model becomes too complex and starts to capture noise in the training data, rather than the underlying patterns. This can lead to poor performance when the model is applied to new, unseen data. To ensure that a model generalizes well to new data, it's important to avoid overfitting. One way to prevent overfitting is by using regularization techniques, which add a penalty to the model's complexity, discouraging it from fitting the noise in the training data. Another approach is to use cross-validation, where the data is split into training and validation sets multiple times to evaluate the model's performance on different subsets of the data. Feature selection is another strategy to prevent overfitting, by reducing the number of input variables to only those that are most relevant for predicting the target variable. This helps simplify the model and reduce the risk of overfitting. Additionally, ensembling techniques, such as bagging and boosting, can help improve the generalization of a model by combining multiple weak learners to create a stronger, more robust model.- The goal of any machine learning model is to generalize well to new, unseen data. By avoiding overfitting and focusing on techniques that promote generalization, you can improve the performance and reliability of your model in real-world applications.
Similar Posts
Continued learning and experimentation are essential for mastering machine learning
To truly master machine learning, it is crucial to emphasize the importance of continued learning and experimentation. Machine ...
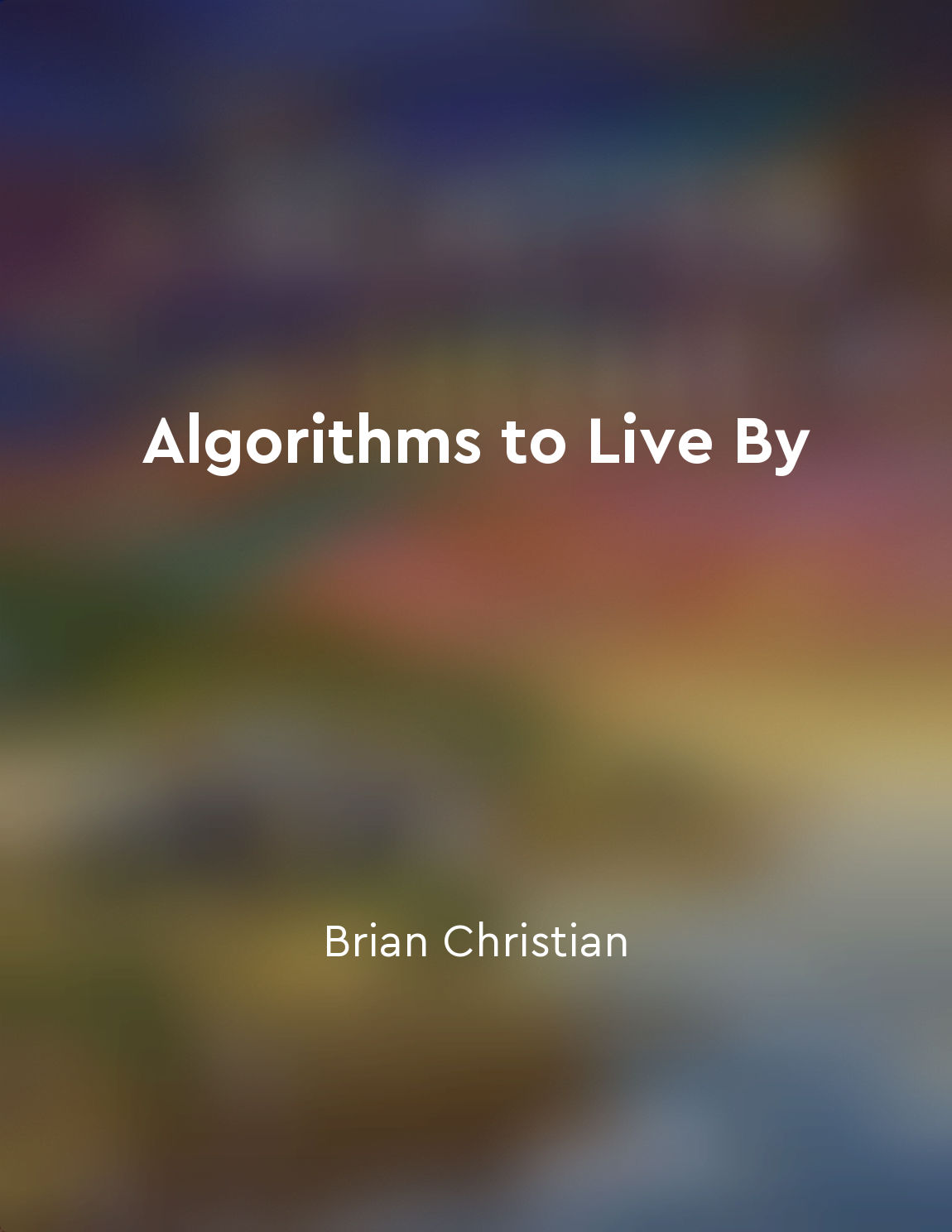
Algorithms are systematic procedures for solving problems
Algorithms serve as systematic procedures for tackling a wide array of problems, offering a structured approach towards finding...
Enhancing communication skills
Improving one's ability to effectively communicate with others is a crucial skill in both personal and professional settings. C...
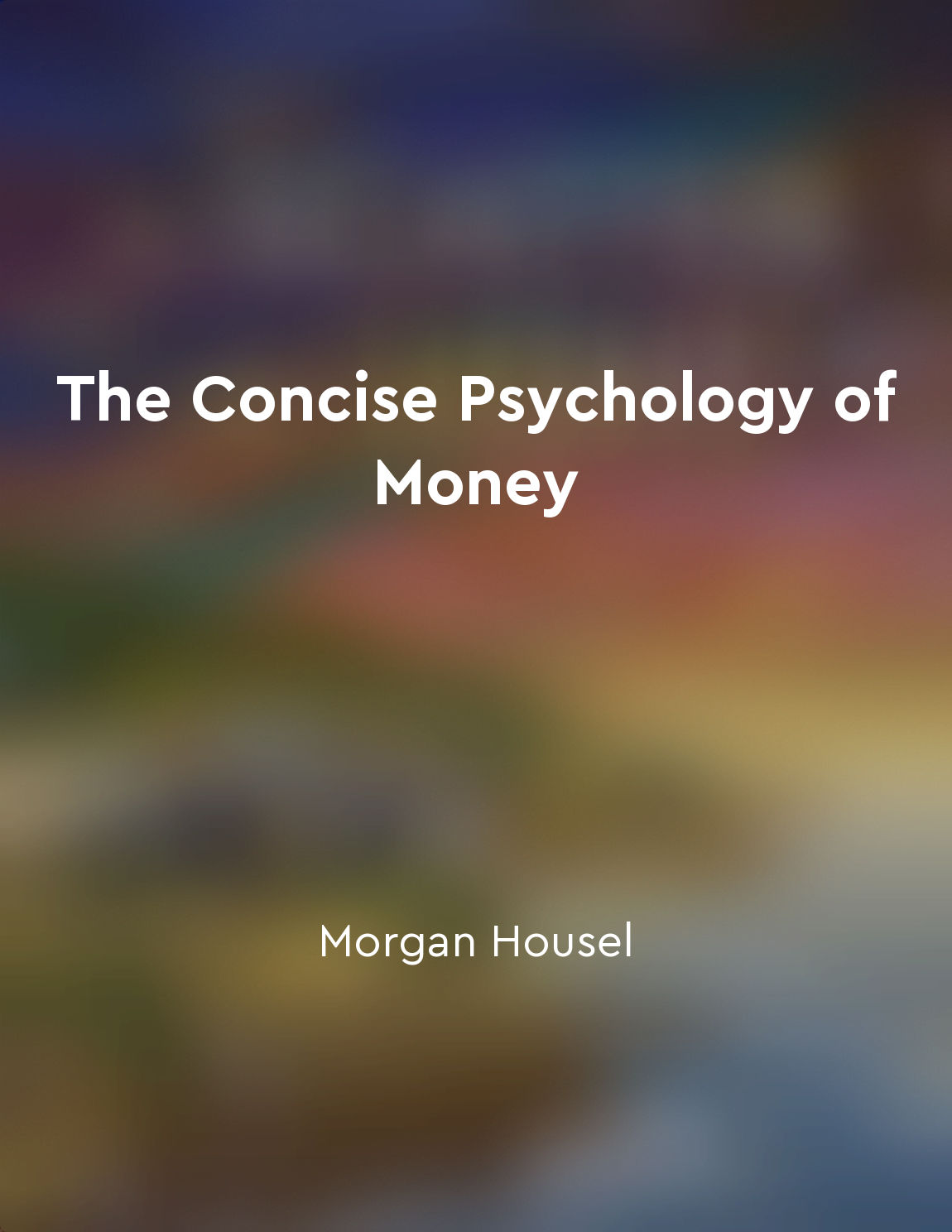
Our financial goals should be aligned with our values and priorities
Our values and priorities shape the decisions we make in every aspect of our lives, including our finances. When it comes to se...
Crossvalidation ensures the generalization of models
Crossvalidation is a technique used to ensure the generalization of models. When we build a model using a training set and then...
Ensemble methods combine multiple models for better performance
Ensemble methods are a powerful approach in machine learning where multiple models are combined to achieve better performance t...
Decision trees break down decisions into a treelike structure
Decision trees are a popular method for classification and regression tasks in machine learning. They break down decisions into...