Audio available in app
Ensemble methods combine multiple models for better predictions from "summary" of Machine Learning For Dummies by John Paul Mueller,Luca Massaron
Ensemble methods are a powerful approach in machine learning that involves combining multiple models to improve overall prediction accuracy. These methods work on the premise that by leveraging the strengths of different models, the weaknesses of individual models can be mitigated. The basic idea behind ensemble methods is to create a diverse set of models that are trained on the same dataset but with different algorithms or subsets of data. By combining the predictions of these diverse models, the ensemble can make more accurate predictions than any single model on its own. There are different types of ensemble methods, such as bagging, boosting, and stacking, each with its own unique approach to combining models. Bagging, for example, involves training multiple models in parallel and aggregating their predictions through a voting mechanism. Boosting, on the other hand, focuses on training models sequentially, with each new model correcting the errors of its predecessor. Ensemble methods are particularly useful when dealing with complex and noisy data, as they can help improve the overall robustness and generalization of the model. By combining multiple models, ensemble methods can also reduce the risk of overfitting, where a model performs well on the training data but poorly on unseen data.- Ensemble methods are a valuable tool in the machine learning toolbox, offering a way to harness the collective intelligence of multiple models for better predictions. Whether you're working on classification, regression, or any other machine learning task, ensemble methods can help improve the accuracy and reliability of your models.
Similar Posts
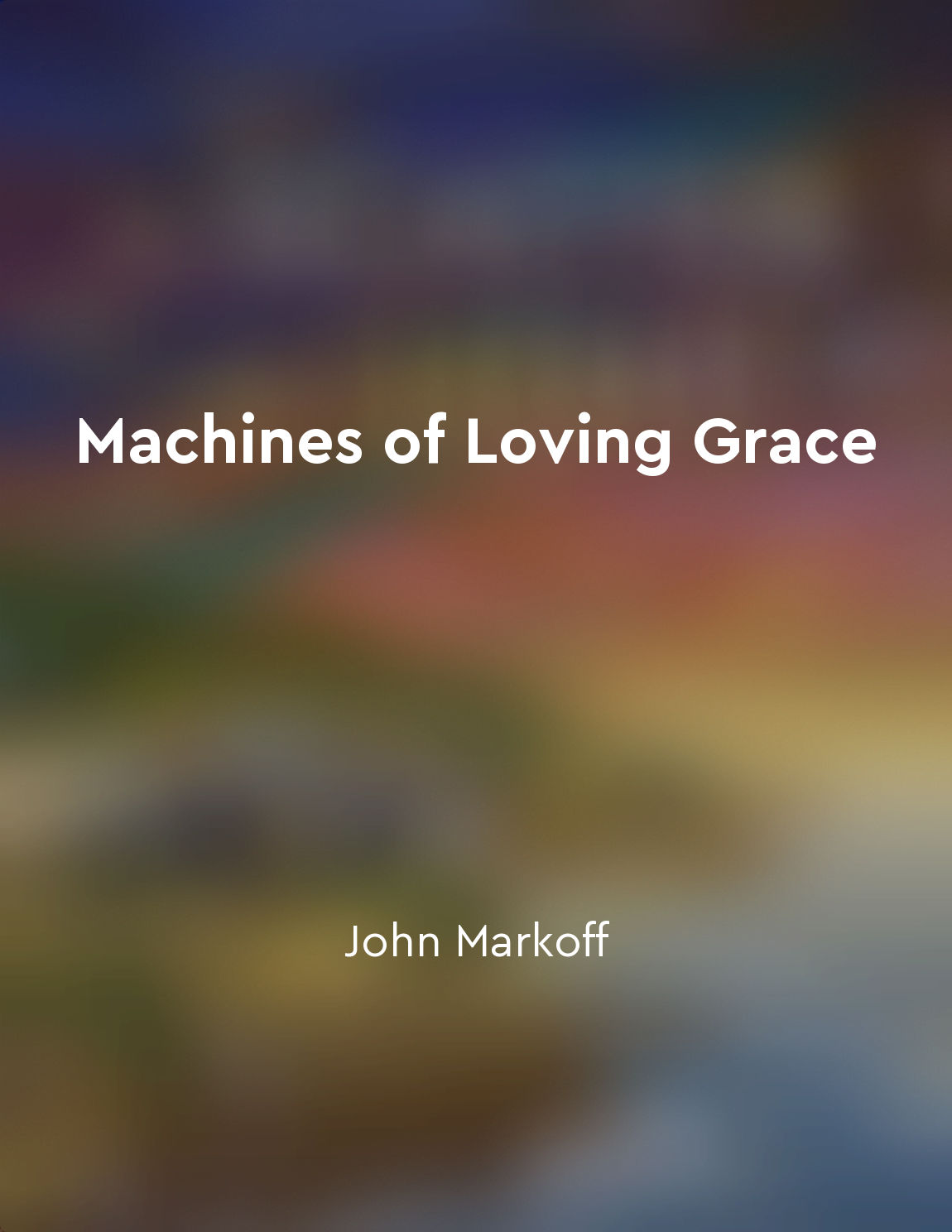
Humans must adapt to the changing technological landscape
Throughout history, humans have faced the challenge of adapting to the ever-changing technological landscape. As new inventions...
Machine learning algorithms help in predicting outcomes
Machine learning algorithms are essential tools for data scientists because they can help predict outcomes based on data patter...
Machine learning utilizes algorithms to make predictions and decisions
Machine learning is a powerful tool that enables computers to learn from data. By utilizing algorithms, machine learning models...
The second law of thermodynamics restricts processes
The second law of thermodynamics plays a fundamental role in dictating the direction in which natural processes can occur. It i...
Overfitting must be avoided to ensure model generalization
Overfitting occurs when a model learns the training data too well, to the point that it starts to memorize the data rather than...
AI technology is advancing rapidly
The speed at which artificial intelligence (AI) technology is progressing is nothing short of astonishing. Innovations are bein...
Data is the fuel for smart machines
Smart machines are powered by data. This is not just any data - it is the lifeblood that fuels these intelligent systems, enabl...
Superforecasters are skilled at recognizing patterns and trends
Superforecasters have a remarkable ability to recognize patterns and trends in the vast sea of information they navigate. They ...
Package your Python code into reusable modules
When you write code in Python, you can put all your functions and variables into a separate file. This file is called a module....