Audio available in app
Different machine learning algorithms have distinct advantages and disadvantages from "summary" of Machine Learning For Dummies by John Paul Mueller,Luca Massaron
When you consider the many different types of machine learning algorithms available to you, it becomes evident that each one has its strengths and weaknesses. For example, some algorithms are better at handling large datasets, while others excel at making accurate predictions. Understanding these distinctions can help you choose the right algorithm for your specific needs. One of the key advantages of using machine learning algorithms is that they can process vast amounts of data quickly and efficiently. This ability allows you to uncover hidden patterns and insights that may not be apparent at first glance. However, not all algorithms are created equal in this regard. Some algorithms may struggle to handle large datasets, leading to slower processing times and potentially less accurate results. Another important consideration when choosing a machine learning algorithm is its ability to generalize from the data. In other words, how well does the algorithm perform when exposed to new, unseen data? Some algorithms may be prone to overfitting, which occurs when the model learns the training data too well and fails to generalize to new data. On the other hand, some algorithms may underfit the data, leading to poor performance on both the training and test datasets. Additionally, the interpretability of a machine learning algorithm is a crucial factor to consider. Some algorithms, such as decision trees and linear regression, are relatively easy to interpret and understand. This transparency can be beneficial when trying to explain the model's predictions to stakeholders. On the other hand, complex algorithms like neural networks may be more challenging to interpret, making them less suitable for certain applications. Furthermore, the computational complexity of an algorithm can also impact its performance. Some algorithms require a significant amount of computational resources to train and evaluate, which may not be feasible in all situations. Additionally, the scalability of an algorithm is essential, as some algorithms may struggle to handle increasingly large datasets or high-dimensional input features.- It's essential to consider the specific characteristics and requirements of your machine learning task when selecting an algorithm. By understanding the distinct advantages and disadvantages of different algorithms, you can make an informed decision that maximizes the performance of your model.
Similar Posts
Crossvalidation helps assess the model's generalization ability
Crossvalidation is a technique used to assess how well a machine learning model can generalize to new, unseen data. When you bu...
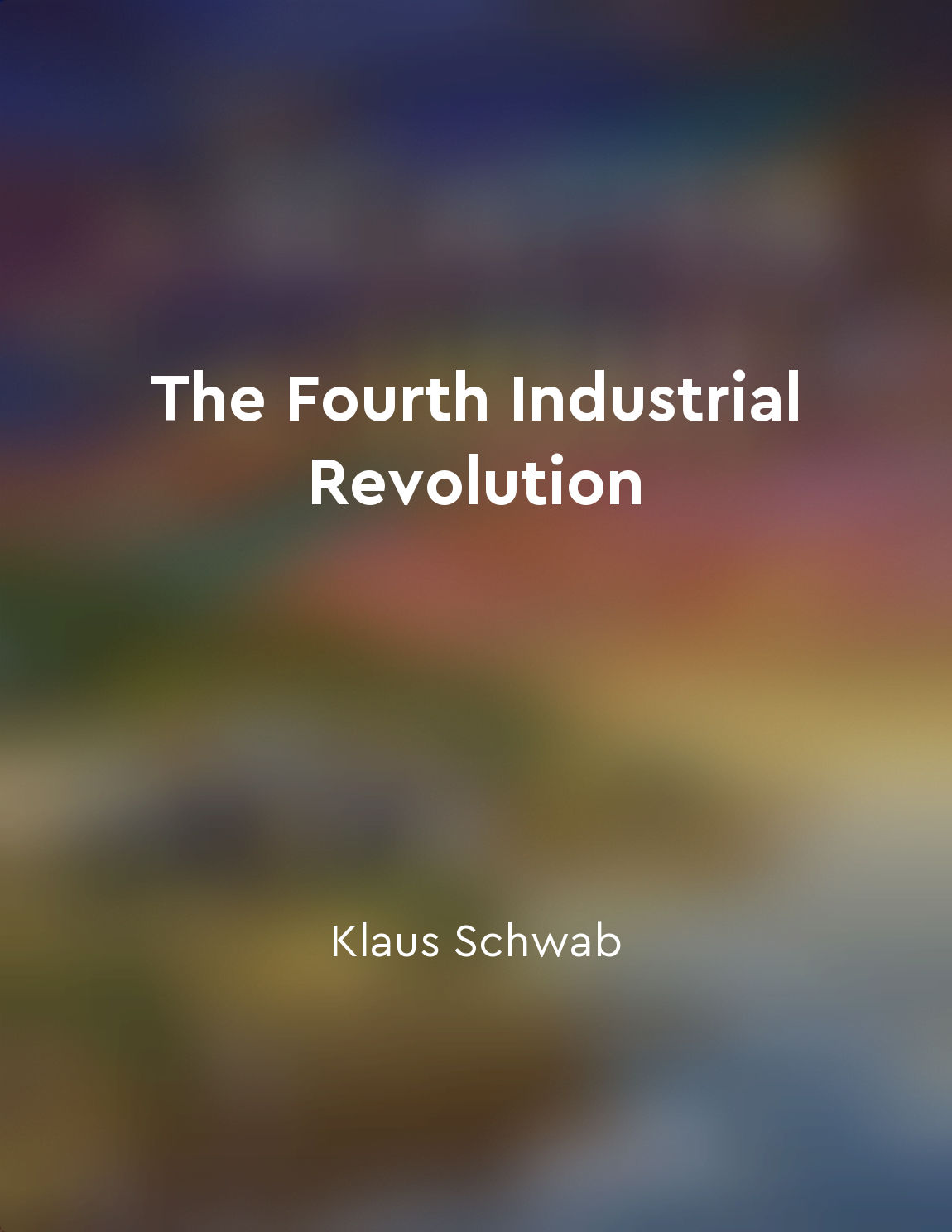
Ethical considerations and regulations are needed to guide the development and deployment of emerging technologies
As we enter the era of the Fourth Industrial Revolution, it is becoming increasingly clear that ethical considerations and regu...
Association rules identify patterns in data sets
Association rules are a powerful tool in data mining that allow us to uncover interesting patterns in large data sets. These ru...
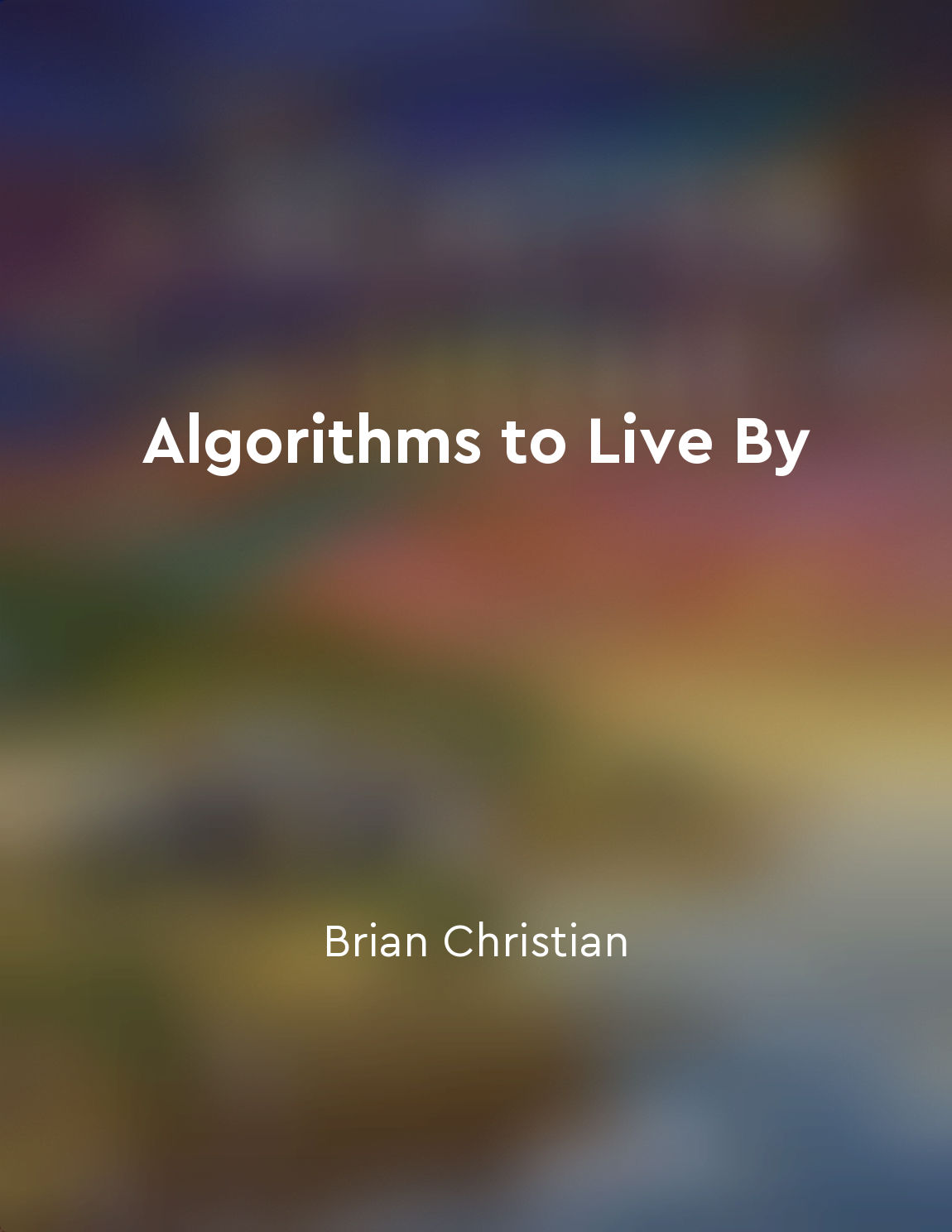
Game theory can help us understand strategic interactions between rational decisionmakers
Game theory offers a framework for understanding how rational decision-makers interact strategically. It provides a way to anal...
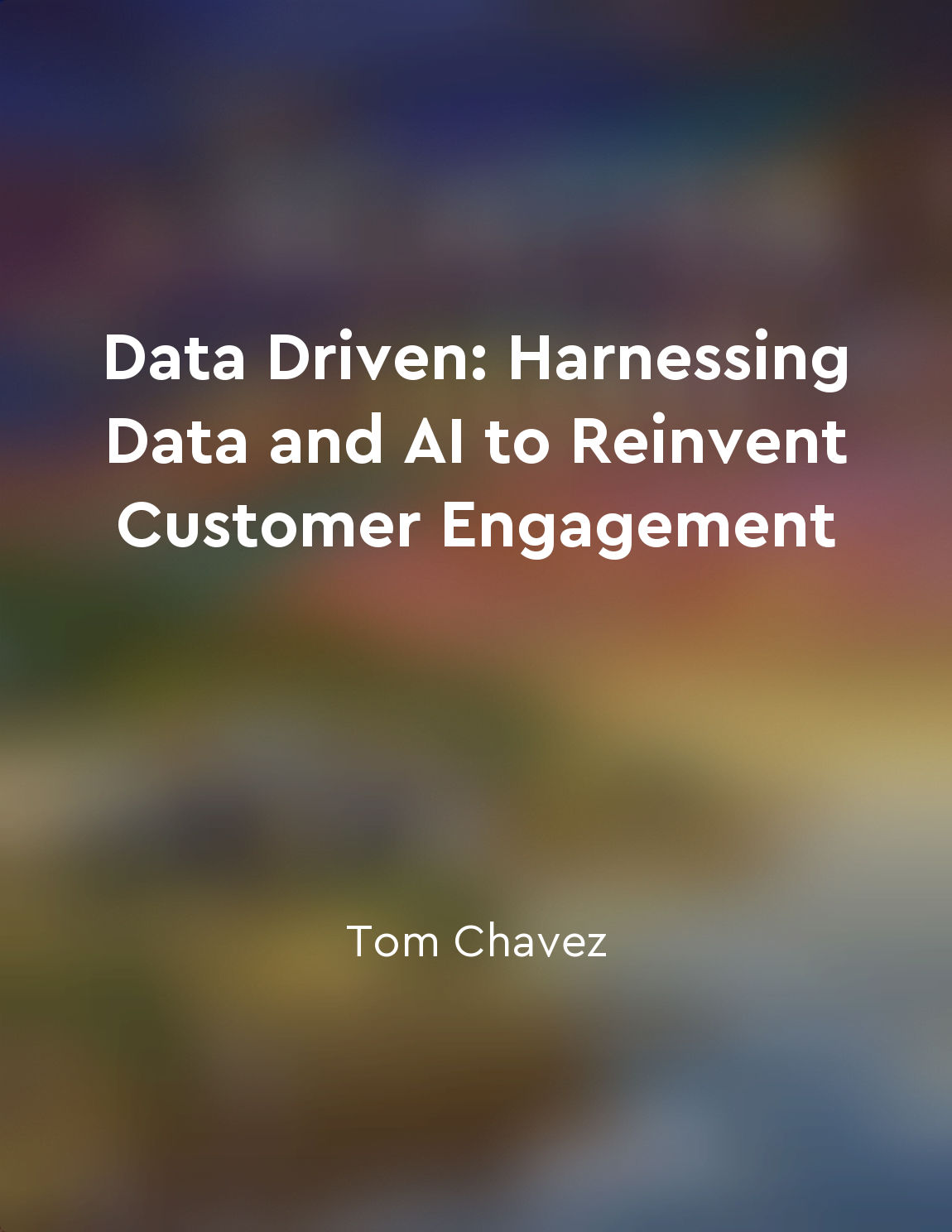
Engagement strategies must evolve
The landscape of customer engagement is constantly changing, driven by advancements in technology, shifts in consumer behavior,...
AI is sparking new ethical debates and challenges
Artificial intelligence is causing quite a stir in the world of ethics. As machines become more capable of making decisions tha...
Decision trees are used for classification and regression tasks
Decision trees are versatile tools that can be used for both classification and regression tasks in machine learning. In classi...