Audio available in app
Overfitting occurs when a model performs well on training data but poorly on new data from "summary" of Data Science for Business by Foster Provost,Tom Fawcett
Overfitting is a common problem faced when training predictive models. It happens when a model becomes too complex and starts to learn the noise in the training data rather than the actual underlying patterns. This can lead to a situation where the model performs exceedingly well on the training data but fails miserably when presented with new, unseen data. In other words, the model has essentially memorized the training data rather than truly learning from it. One way to understand overfitting is to think of it as fitting the training data too closely, to the point where the model starts to capture the random fluctuations and outliers in the data. These fluctuations and outliers are unique to the training data and are not representative of the general patterns that the model should be learning. As a result, when the model is tested on new data, it struggles to generalize beyond the specific quirks of the training set. Overfitting can be detrimental to the performance of a predictive model because it compromises its ability to make accurate predictions on unseen data. This is a critical issue in data science because the ultimate goal is to build models that can generalize well to new, unseen data and provide valuable insights or predictions. When a model overfits, it becomes unreliable and loses its predictive power, making it practically useless for real-world applications. To prevent overfitting, data scientists employ various techniques such as cross-validation, regularization, and feature selection. These techniques help to simplify the model and reduce the risk of it memorizing the noise in the training data. By striking a balance between complexity and generalization, data scientists can build models that not only perform well on the training data but also generalize effectively to new, unseen data. This is crucial for ensuring the reliability and usefulness of predictive models in practical applications.Similar Posts
Postindustrial society
In the broadest terms, the postindustrial society is one in which the majority of the workforce is no longer engaged in making ...
Natural language processing enables machines to understand human language
Natural language processing (NLP) is a subfield of artificial intelligence that focuses on enabling machines to understand and ...
Feature selection plays a key role in model performance
When you're working on a machine learning project, selecting the right features is crucial for the overall performance of your ...
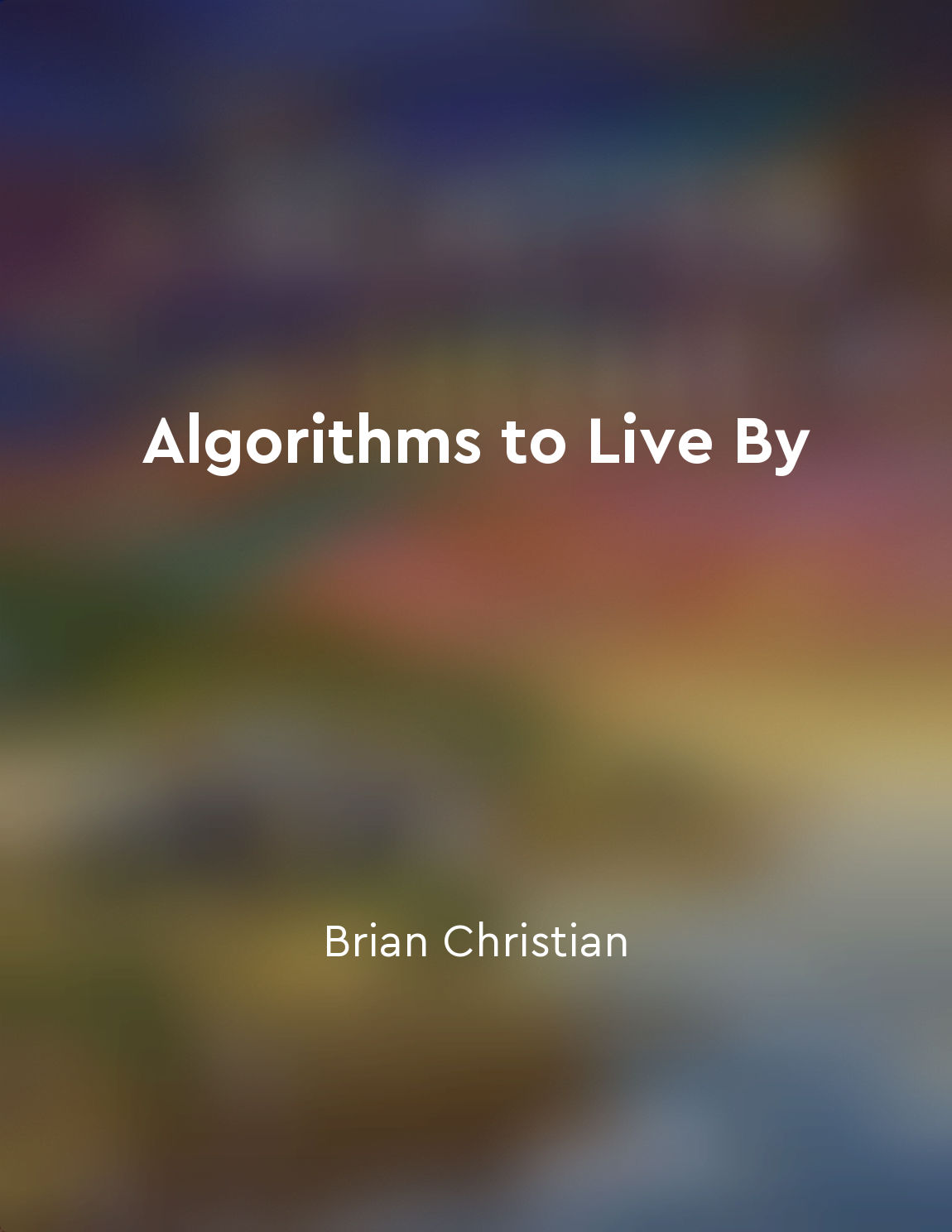
Caching can help us store and retrieve information quickly
Imagine you're at a library, searching for a book on a topic that has piqued your interest. Instead of having to go through the...