Audio available in app
Neural networks are a powerful tool for modeling complex relationships in data from "summary" of Data Science for Business by Foster Provost,Tom Fawcett
Neural networks have gained popularity in data science due to their ability to capture complex relationships within data. These powerful tools are capable of learning intricate patterns and structures in the data, making them highly effective for modeling a wide range of problems. By mimicking the way the human brain processes information, neural networks can handle nonlinear relationships and interactions that may be challenging for traditional statistical models to capture. The strength of neural networks lies in their architecture, which consists of interconnected nodes organized in layers. Each node processes information and passes it on to the next layer, allowing for the extraction of features at different levels of abstraction. This hierarchical structure enables neural networks to learn from data and make predictions based on the relationships they uncover. In essence, neural networks excel at understanding complex data patterns by leveraging their interconnected layers of computation. Moreover, neural networks can be trained on large datasets to recognize intricate patterns that may not be immediately apparent to human analysts. Through a process known as backpropagation, neural networks adjust their weights and biases to minimize errors and improve their predictive accuracy. This iterative learning process allows neural networks to continually refine their models and enhance their ability to capture complex relationships in the data. In practical terms, neural networks are used in a wide range of applications, from image and speech recognition to natural language processing and financial forecasting. Their versatility and adaptability make them well-suited for solving complex problems across various industries. As data continues to grow in volume and complexity, neural networks offer a valuable tool for extracting insights and making informed decisions based on the intricate relationships present in the data.Similar Posts
The future of AI is uncertain but holds great promise for humanity
The path ahead for artificial intelligence (AI) is shrouded in uncertainty, yet it brims with immense potential for humanity. A...
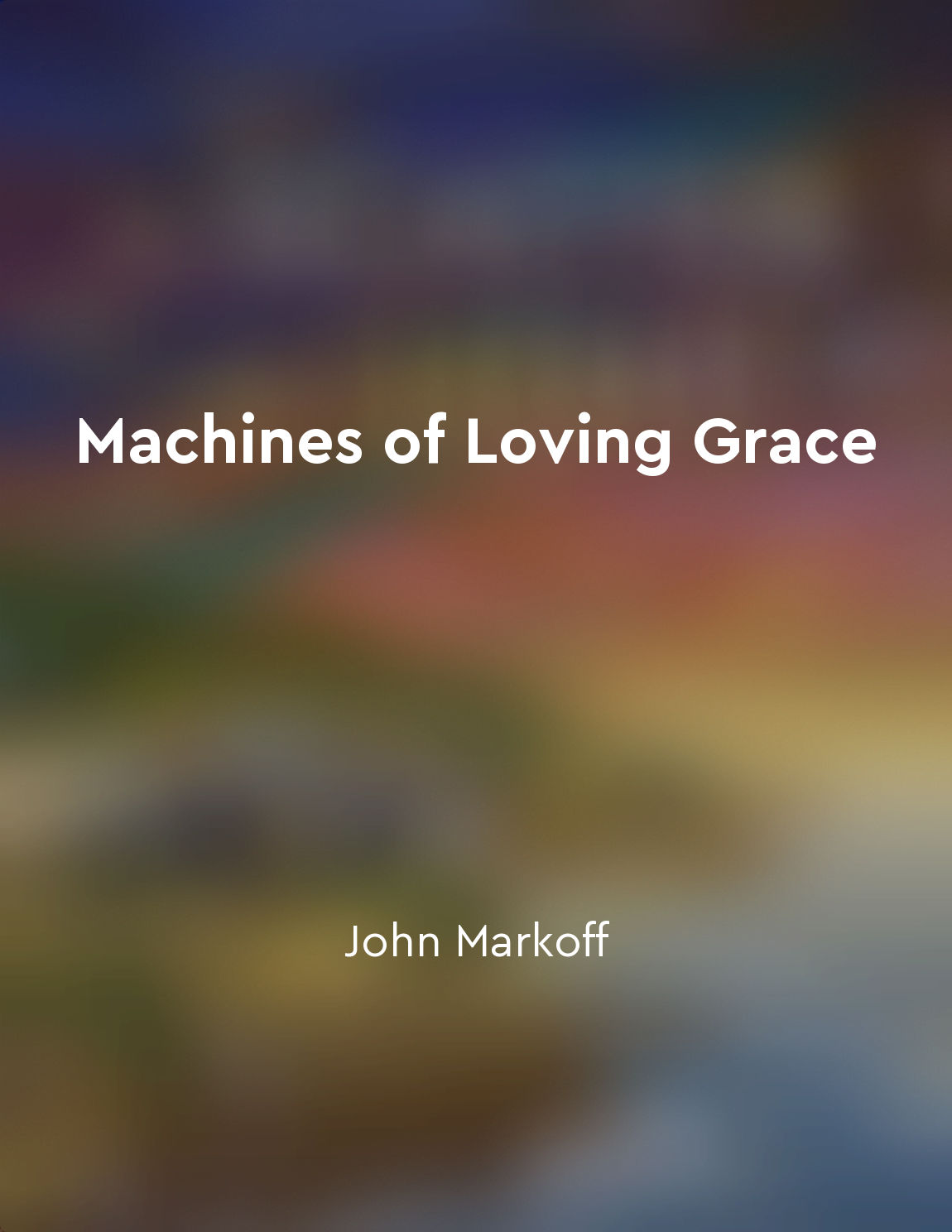
The potential for AI is vast
The boundless potential of artificial intelligence stretches out before us like an uncharted wilderness, waiting to be explored...
Grace Hopper popularized highlevel programming languages
Grace Hopper played a pivotal role in the development and popularization of high-level programming languages. Prior to her cont...
Variables store data
When we write a program, we often need to keep track of information. We use variables to store this information. A variable is ...