Audio available in app
Evaluation metrics are used to assess model performance from "summary" of Data Science for Business by Foster Provost,Tom Fawcett
Evaluation metrics play a crucial role in the data science process by providing a way to measure how well a model is performing. These metrics are essential in determining the effectiveness of a model in solving the specific problem it was designed for. By evaluating the performance of a model using these metrics, data scientists can gain insights into its strengths and weaknesses, which can then be used to make improvements. There are various evaluation metrics that can be used to assess model performance, depending on the nature of the problem being solved. For example, in a classification problem, metrics such as accuracy, precision, recall, and F1 score can be used to evaluate how well the model is classifying the data. On the other hand, in a regression problem, metrics such as mean squared error, mean absolute error, and R-squared can be used to assess the model's ability to predict continuous values. It is important to choose the right evaluation metrics based on the specific goals of the project. For instance, if the goal is to minimize false positives, precision may be a more important metric to consider. On the other hand, if the goal is to capture as many true positives as possible, recall may be a more relevant metric. By selecting the appropriate evaluation metrics, data scientists can ensure that they are measuring the performance of the model in a way that aligns with the project objectives. In addition to choosing the right evaluation metrics, it is also important to consider the context in which the model will be deployed. For example, if a model is being used in a medical setting to predict the likelihood of a disease, false negatives may have more severe consequences than false positives. In such cases, the evaluation metrics should be weighted accordingly to reflect the relative importance of each type of error.- Evaluation metrics are a critical component of the data science process, providing a way to quantify and measure the performance of a model. By selecting the appropriate metrics and considering the context in which the model will be used, data scientists can gain valuable insights into how well their model is performing and make improvements as needed.
Similar Posts
Data scientists use Python and R for analysis
Data scientists rely heavily on programming languages like Python and R to carry out their data analysis tasks. These languages...
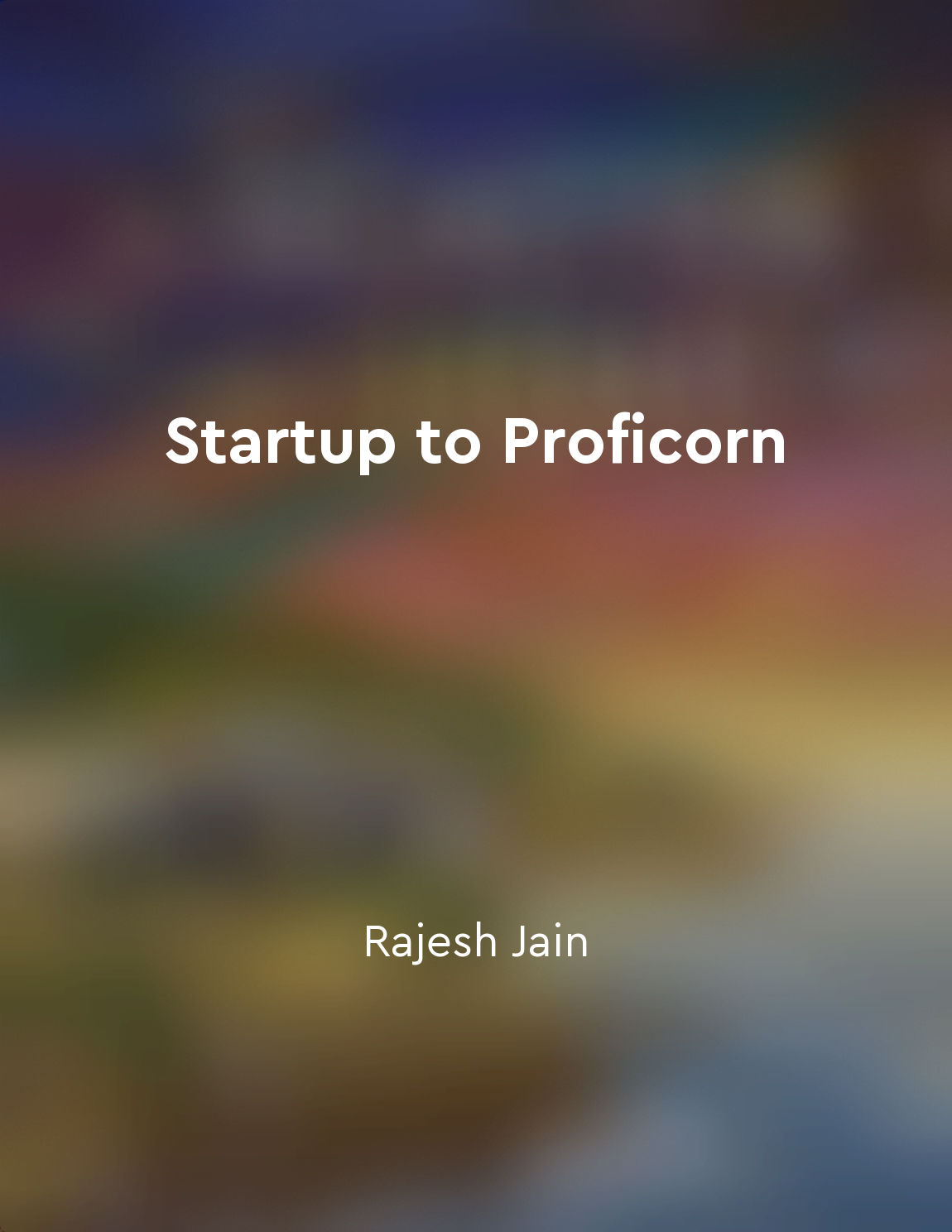
Automate and scale processes for efficiency
To build a proficorn, it is essential to focus on automating and scaling processes for efficiency. This involves leveraging tec...

Mobile apps improve health outcomes
Mobile apps have emerged as a powerful tool in the realm of healthcare, offering the promise of improving health outcomes in a ...
Understanding user motivations informs design choices
To create effective experiences, it is crucial to understand the motivations that drive users. By delving into the reasons behi...
Python is a popular programming language
Python has gained immense popularity in recent years for a variety of reasons. One of the key factors contributing to its wides...
Python is a versatile language used in various industries
Python is a versatile language that is widely used across various industries due to its simplicity and ease of learning. Its cl...
Big data fuels predictive analytics
The vast expanse of big data serves as the driving force behind predictive analytics. This data is the lifeblood that powers th...
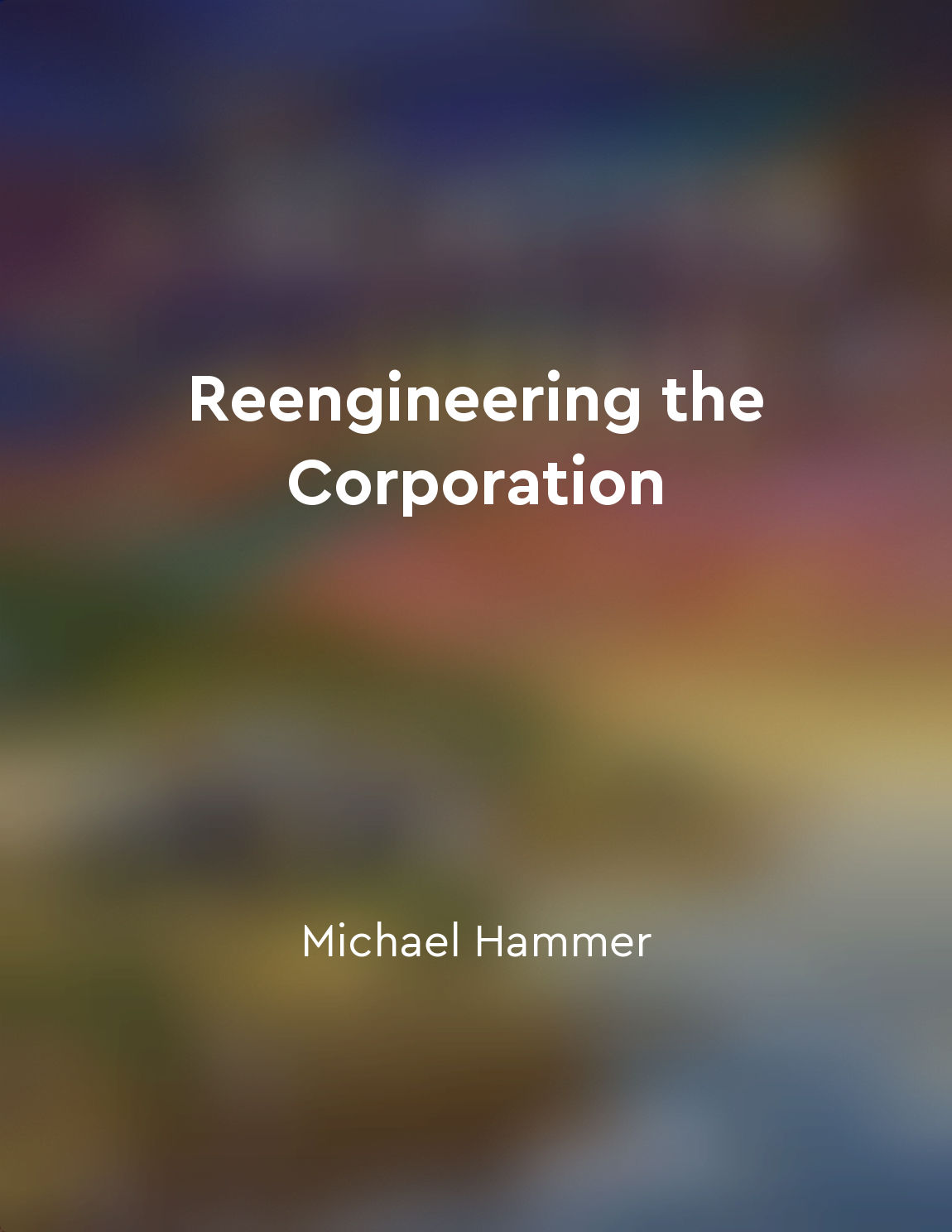
Reengineering involves radically redesigning processes for improved performance
Reengineering goes beyond making incremental improvements to existing processes. It demands a complete overhaul of the way work...