Audio available in app
Bias and variance tradeoff is important for finding the right balance in model performance from "summary" of Data Science for Business by Foster Provost,Tom Fawcett
Bias and variance are two sources of error in the prediction of machine learning models. Bias is error caused by the simplifying assumptions made by a model. A high-bias model may oversimplify the data and fail to capture important patterns, leading to underfitting. On the other hand, variance is error caused by the model's sensitivity to fluctuations in the training data. A high-variance model may capture noise in the training data and fail to generalize well to new, unseen data, leading to overfitting. The bias-variance tradeoff is the balance between these two sources of error. A model with high bias tends to have low variance, while a model with high variance tends to have low bias. Finding the right balance between bias and variance is crucial for achieving optimal model performance. If a model has high bias, it may be too simple to capture the complexity of the data, resulting in poor predictive performance. On the other hand, if a model has high variance, it may be too sensitive to the training data, resulting in poor generalization to new data. To find the right balance between bias and variance, it is important to understand the tradeoff between the two. Increasing the complexity of a model typically reduces bias but increases variance, while decreasing the complexity of a model typically reduces variance but increases bias. By tuning model complexity, one can find the optimal balance between bias and variance that maximizes predictive performance. Regularization techniques, such as L1 and L2 regularization, can help control the bias-variance tradeoff by penalizing overly complex models. Cross-validation can also be used to estimate a model's bias and variance and select the best model based on predictive performance. By carefully managing the bias-variance tradeoff, one can develop models that generalize well to new data and make accurate predictions.Similar Posts
The internet can amplify cognitive biases
In our digital age, the internet has become a powerful tool for accessing information and connecting with others. However, this...
Collaboration between humans and AI is key to success
The key to success in the future will undoubtedly be the collaboration between humans and artificial intelligence. This partner...
The ethical use of smart machines is essential for society
The integration of smart machines into society brings about a myriad of benefits, from enhancing efficiency to improving qualit...
Data governance ensures data quality and security
Data governance is a critical component of any organization's data strategy. It involves the creation and enforcement of polici...
Files can be read and written in Python
Reading and writing files is a crucial aspect of any programming language, including Python. In Python, you can easily open, re...
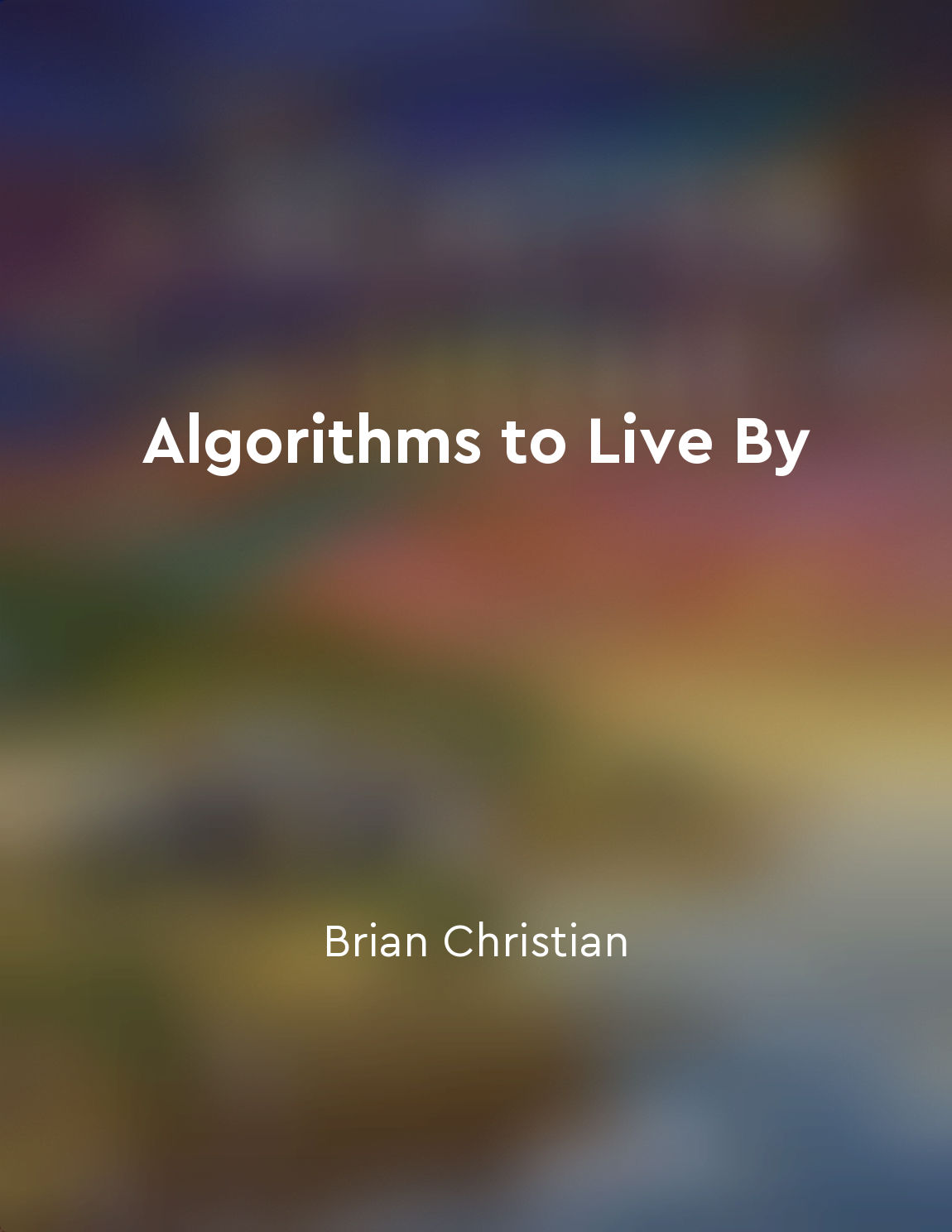
Algorithms can provide us with a framework for making better decisions in a variety of situations
Algorithms offer us a valuable tool for navigating the complexities of decision-making in our daily lives. By breaking down a p...
Embrace the power of predictive analytics
To fully grasp the concept of predictive analytics, one must come to terms with its inherent power. It is not merely a tool or ...
AI has the ability to predict trends and behaviors with accuracy
One of the remarkable capabilities of artificial intelligence is its power to forecast trends and behaviors with remarkable pre...
Digital networks
Digital networks are, in the abstract, nothing more than a means of conveying information from one point to another. They are t...
Data science is essential for making informed business decisions
Data science plays a crucial role in helping businesses make informed decisions. By analyzing data, businesses can gain valuabl...