Audio available in app
Evolutionary algorithms mimic the process of natural selection to optimize solutions from "summary" of Artificial Intelligence by Melanie Mitchell
Evolutionary algorithms are a class of optimization algorithms that draw inspiration from the process of natural selection. The main idea behind these algorithms is to simulate the process of evolution in order to find the best solution to a given problem. Just as in natural selection, where individuals with favorable traits are more likely to survive and reproduce, in evolutionary algorithms, solutions that perform well on a given task are more likely to be selected for further exploration. The process starts with generating a population of candidate solutions to the problem at hand. These solutions are evaluated based on a fitness function, which quantifies how well each solution performs on the task. The solutions with higher fitness values are then selected to "reproduce" and create offspring solutions. This reproduction process involves recombining and mutating the selected solutions to generate new candidate solutions. Through successive generations of selection, reproduction, and mutation, evolutionary algorithms drive the population towards better and better solutions. Over time, the algorithm converges towards an optimal or near-optimal solution to the problem. This process of iteratively improving solutions through simulated evolution is what allows evolutionary algorithms to optimize solutions effectively. One of the key advantages of evolutionary algorithms is their ability to search large and complex solution spaces efficiently. By exploring a diverse set of candidate solutions and leveraging the principles of natural selection, these algorithms can effectively navigate through rugged and high-dimensional search spaces to find good solutions. This makes evolutionary algorithms particularly well-suited for optimization problems where traditional search algorithms may struggle.- Evolutionary algorithms provide a powerful and flexible approach to optimization that draws inspiration from the natural world. By mimicking the process of natural selection, these algorithms are able to efficiently optimize solutions to a wide range of problems across different domains.
Similar Posts
Businesses are leveraging smart machines to gain a competitive edge
In the hyper-competitive landscape of modern business, companies are turning to smart machines as a way to distinguish themselv...
Worry
Worry is a state of mind that often arises from thinking about the things that could go wrong in the future. It is a negative e...
The precautionary principle should guide the regulation of genetically modified crops
The precautionary principle, when applied to the regulation of genetically modified crops, dictates that the potential risks an...
If statements control program flow
When writing a program, one of the most important tools in your toolbox is the ability to make decisions based on certain condi...
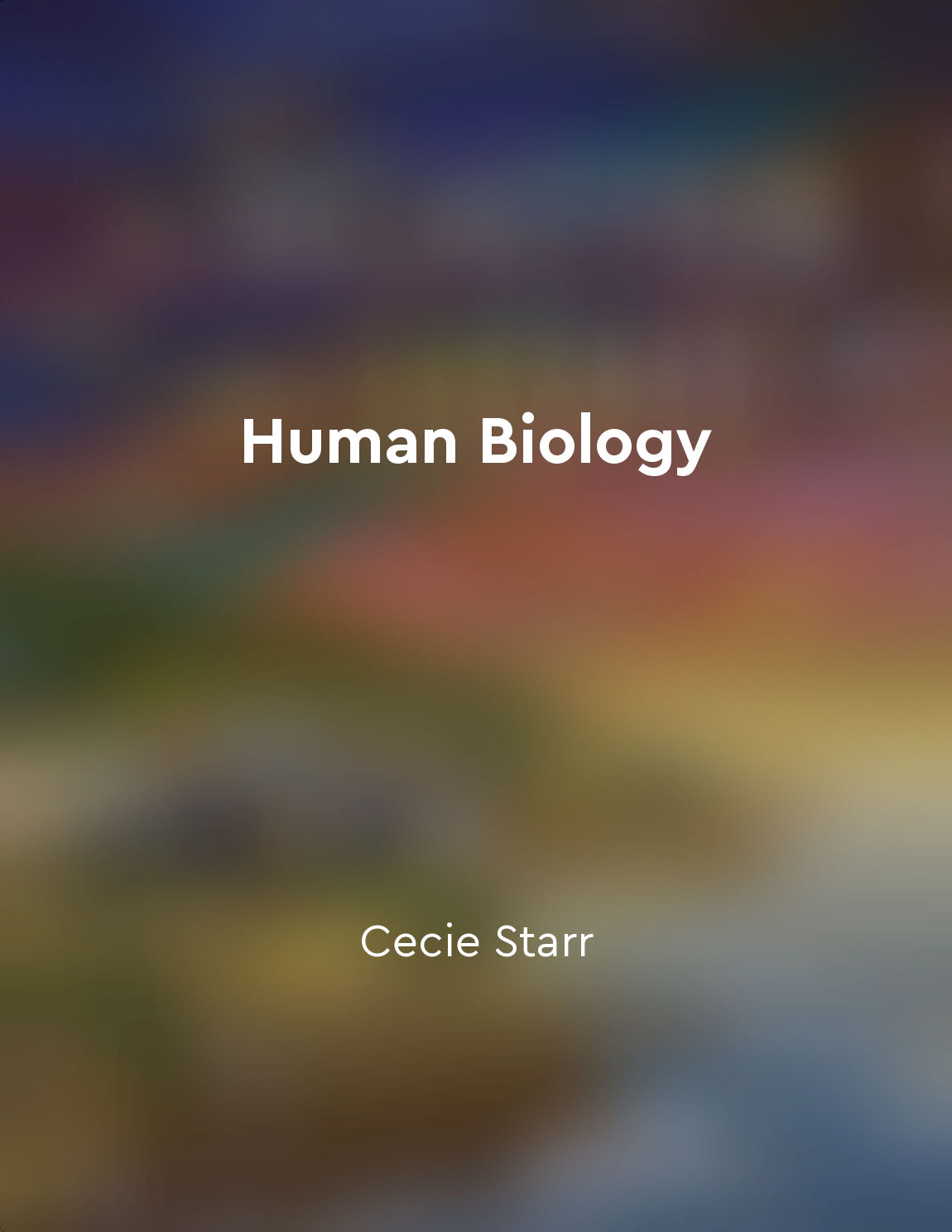
The nervous system coordinates body functions
The nervous system plays a crucial role in coordinating the various functions of the body. This complex system is responsible f...
Humans and machines can form symbiotic relationships
The bond between humans and machines goes beyond mere interaction; it can evolve into a symbiotic relationship that benefits bo...
System dynamics involve nonlinear relationships
System dynamics involve nonlinear relationships, which means that the behavior of a system does not change in proportion to the...
Criticism is essential for progress
Criticism is not just beneficial for progress; it is essential. It is through criticism that we are able to identify errors and...